Where's the Vaccine against AI Hype?
Moderna x OpenAI = Best Case, a Nothingburger
By Emily
I don't do predictions, but I do spend a good bit of time these days pondering how things might play out when the current AI hype bubble finally pops, or slowly deflates, or drifts away, or whatever it does. It seems clear by now that the engine keeping this hot air balloon (if I can abruptly switch to a nearby metaphor) inflated and afloat is the frothy interest of venture capitalists and the finance side of Big Tech. But that air has been blowing so hot and so long now that its miasma has long since infected just about every other sector. And I worry that when the VCs start focusing somewhere else, we'll still be stuck digging out from entrenched surveillance as well as business processes that have been contorted to jump on the ChatGPT bandwagon.
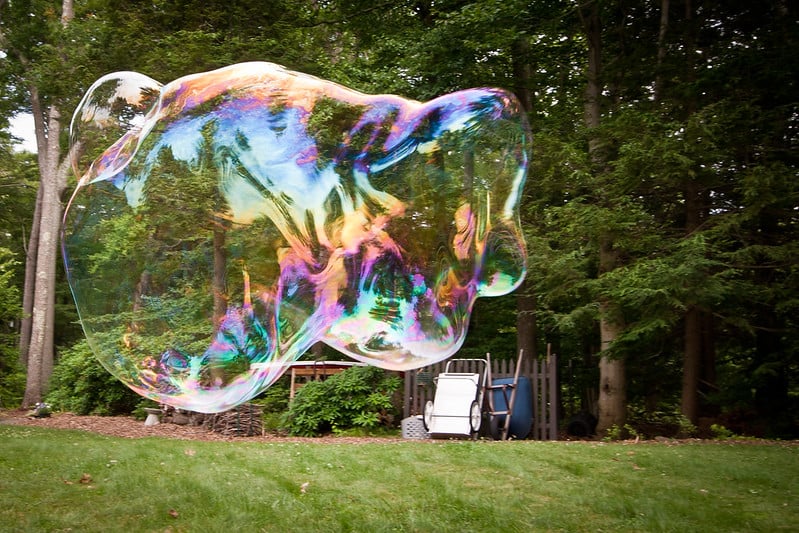
As a case in point, last Monday (April 29), the Wall Street Journal reported on a new partnership between OpenAI and Moderna. Before I go further, let me say that I write with deep gratitude to the scientists at Moderna and everyone else who contributed to the development and roll-out of COVID vaccines. And so it is even more disheartening to read about Moderna jumping on this bandwagon.
The article is full of hype-y statements from Sam Altman (the subhead reads "People literally talk about how AI is going to cure diseases someday, and I think this is a very meaningful first step") and big numbers ("Moderna employees have created over 750 unique, tailored versions of OpenAI’s ChatGPT, also known as GPTs, that are designed to facilitate specific tasks or processes across the business"), but very thin on specifics.
And the specifics that are available are ... alarming to say the least. Here are the use cases that are discussed in any detail:
1. Choosing doses for clinical trials
Quoted from the WSJ article:
Of the 750 GPTs built until now, one uses years of previous research and medical knowledge to predict the optimal dose of a drug for clinical trials. Dose optimization is a huge challenge, and choosing the wrong dose can result in products being killed in the clinical-trial stage.
It seems very plausible to me that statistical analysis over previous clinical trials could be informative for narrowing down doses to explore. But I would also hope that the scientists at Moderna have a way to do this that is knowledge-driven. What are the variables of interest? If that information isn't already available as structured data, how do you take the available information and reliably create a database of it? What are the appropriate statistical tests to run over the data?
But the article doesn't describe that, only describing "tailored versions of ChatGPT", which leads me to believe that they've maybe fine-tuned ChatGPT on research reports about clinical trials and written some form of prompt that gets it to spit out information about drug doses for hypothetical future trials. To anyone who understands that ChatGPT is effectively a glorified Magic 8 Ball, the absurdity of this is obvious. But given OpenAI's track record (Altman's statements made within this article, and other cases, like Ilya Sutskever leading employees in a chant of "feel the AGI!"), I'm afraid they don't see the absurdity -- and that the folks at Moderna have been taken in.
Indeed, the WSJ quotes Moderna CEO Stéphane Bancel as saying that "he’s been obsessed with ChatGPT since Christmas of 2022, and it’s one of four apps at the bottom of his iPhone, along with the apps for phone, text and internet. His goal is for employees to use it no fewer than 20 times a day, and so far, they’re keen, he said."
2. Predicting enzyme structure
Quoting further:
Another one, on the drug manufacturing side, is helping predict the structure of new enzymes that will enable manufacturing processes with better yield and reduced waste.
This is the entirety of the info available on this one in the article, so it's hard to know what's going on here, but I'd start with my standard questions: What is the training data for the GPT? What is the input at runtime? What is the output? What's being done with that output? How is that output integrated into existing processes? What are the failure modes, and what are their consequences?
3. Answering regulator's questions
Another GPT combs through swaths of research to draft answers to questions from regulators—turning what used to be a weeks-long process into something that can happen in minutes, Bancel said.
And this is just appalling. Pharmaceuticals are highly regulated for a reason, and for a well-respected company to brag about taking just minutes to answer questions from regulators is frankly frightening. I realize this says that it's about the process of drafting answers, but how is that information being verified? What's happening to those drafts? I assume the process took weeks because it was about gathering and checking information. You know, details that are important, being reported to a regulatory body making sure the products are safe for the public. No matter what post-editing happens, starting from the output of a synthetic text extruding machine that will be very good at making things "sound good" is simply not appropriate. I hope the FDA is paying attention.
Our book, The AI Con, is now available wherever fine books are sold!
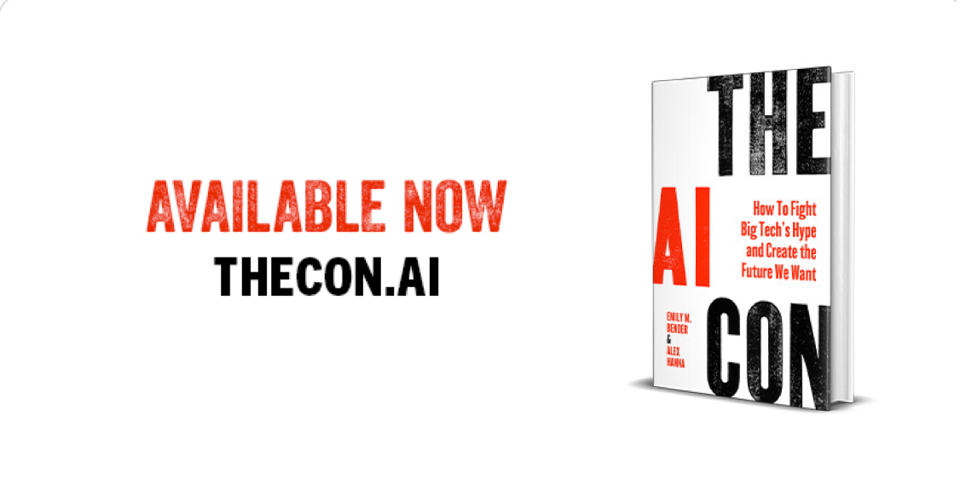