On the energy demands of "AI"
Big Tech's Tempting Promises of Carbon Zero are Hollow
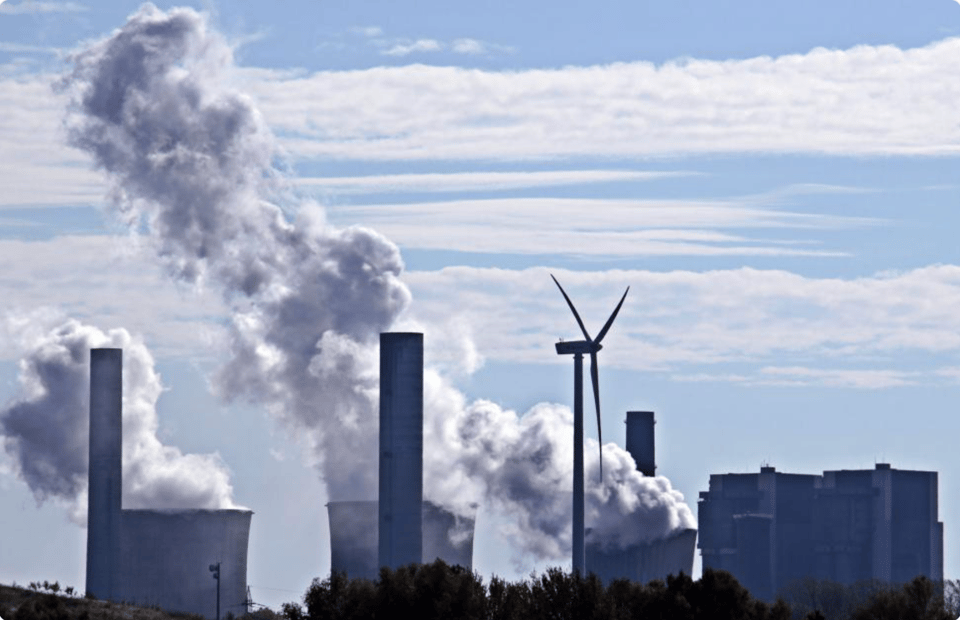
By Emily
tl;dr
Researchers have been sounding the alarm about the carbon footprint and other environmental impacts of the large machine learning models (language models, text-image models, audio, video, etc.) for at least five years now. Meanwhile, many of the tech companies building and selling these models are reassuring us that they're on the road to net zero carbon, even while building ever more data centers. Recent reporting in the Washington Post documents how those data centers are leading utility companies to burn more fossil fuels.
In a bit more detail
In 2019, at the 57th annual meeting of the Association for Computational Linguistics (held in Florence, Italy), Emma Strubell and co-authors presented a paper alerting the field to the energy usage and associated carbon footprint of large-scale experiments with language models and similar technology:
In this paper we bring this issue to the attention of NLP researchers by quantifying the approximate financial and environmental costs of training a variety of recently successful neural network models for NLP. Based on these findings, we propose actionable recommendations to reduce costs and improve equity in NLP research and practice.
They further point out (emphasis added):
Though some of this energy may come from renewable or carbon credit-offset resources, the high energy demands of these models are still a concern since (1) energy is not currently derived from carbon-neutral sources in many locations, and (2) when renewable energy is available, it is still limited to the equipment we have to produce and store it, and energy spent training a neural network might better be allocated to heating a family’s home.
Also in 2019, at the Workshop on Tackling Climate Change with Machine Learning at the Conference on Neural Information Processing Systems (NeurIPS 2019), Alexandre Lacoste, Sasha Luccioni, Victor Schmidt, and Thomas Dandres presented a paper and a "machine learning emissions calculator" to enable researchers to quantify the emissions of their models. They wrote:
While a decade ago, only a few ML pioneers were training neural networks on GPUs (Graphical Processing Units), in recent years powerful GPUs have become increasingly accessible and used by ML practitioners worldwide. Furthermore, new models often need to beat existing challenges, which entails training on more GPUs, with larger datasets, for a longer time. This expansion brings with it ever-growing costs in terms of the energy needed to fuel it.
The following year, Alan Borning, Batya Friedman, and Nick Logler published "The ‘Invisible’ Materiality of Information Technology" in Communications of the ACM. They write:
One important metaphor here is that of “cloud computing,” which conjures up images of something light and insubstantial, floating up in the sky. This metaphor highlights that the servers and their supporting infrastructure are located someplace else, and that users of the cloud need not concern themselves with how they are maintained, monitored, powered, cooled, and so forth; it tends to hide that they are even material at all.
In 2021, in the Stochastic Parrots paper, presented at the Conference on Fairness Accountability and Transparency, we wrote:
[...] renewable energy sources are still costly to the environment, and data centers with increasing computation requirements take away from other potential uses of green energy, underscoring the need for energy efficient model architectures and training paradigms.
and:
When we perform risk/benefit analyses of language technology, we must keep in mind how the risks and benefits are distributed, because they do not accrue to the same people. On the one hand, it is well documented in the literature on environmental racism that the negative effects of climate change are reaching and impacting the world’s most marginalized communities first. Is it fair or just to ask, for example, that the residents of the Maldives (likely to be underwater by 2100) or the 800,000 people in Sudan affected by drastic floods pay the environmental price of training and deploying ever larger English LMs, when similar large-scale models aren’t being produced for Dhivehi or Sudanese Arabic?
That's only a sampling of the work in this area. There is plenty more!
The Stochastic Parrots paper became famous in large part because it upset someone inside of Google (even though the running example was OpenAI's GPT-3, not anything from Google)—and two of the authors were ultimately fired over it.
In early 2021, researchers at UC Berkeley and Google (David Patterson, Joseph Gonzalez, Quoc Le, Chen Liang, Lluis-Miquel Munguia, Daniel Rothchild, David So, Maud Texier, Jeff Dean) posted a paper to arXiv entitled "Carbon Emissions and Large Neural Network Training". They eventually published a similar paper as "The Carbon Footprint of Machine Learning Training Will Plateau, Then Shrink" in IEEE Explore (2022).
In this paper, the authors take issue with how Strubell et al. estimated the impact of a specific model, on the grounds that Strubell et al. used estimates that didn't match the actual energy mix, processors, and experimental settings—actually just underscoring Strubell et al.'s arguments about the necessity for transparency about energy usage. They also argue (with some hedging):
Another perspective is that some consider the carbon footprint to be erased entirely if a cloud provider matches 100% of its energy consumption with renewable energy, as Google and Facebook have done and as Microsoft will soon do. [IEEE 2022 version.]
And indeed, Google, Meta, and Microsoft have all made big promises about getting to net-zero carbon emissions.
So, how's that working out? Well, as Strubell and colleagues pointed out in 2019 and others (including the Stochastic Parrots authors) have reiterated since, it turns out that, added energy demand means added energy demand. If you use clean energy sources for that added demand, you've used up some of the clean energy supply that could have gone somewhere else. Until we actually have more clean energy than we need, throwing lots of it at training and using "generative AI" models is just going to make the climate crisis worse.
Evan Harper and Caroline O'Donovan, reporting in The Washington Post on June 21, 2024, have some alarming updates:
In the Salt Lake City region, utility executives and lawmakers scaled back plans for big investments in clean energy and doubled down on coal. The retirement of a large coal plant has been pushed back a decade, to 2042, and the closure of another has been delayed to 2036.
A spike in tech-related energy needs in Georgia moved regulators in April to green-light an expansion of fossil fuel use, including purchasing power from Mississippi that will delay closure of a half-century-old coal plant there. In the suburbs of Milwaukee, Microsoft’s announcement in March that it is building a $3.3 billion data center campus followed the local utility pushing back by one year the retirement of coal units, and unveiling plans for a vast expansion of gas power that regional energy executives say is necessary to stabilize the grid amid soaring data center demand and other growth.
In Omaha, where Google and Meta recently set up sprawling data center operations, a coal plant that was supposed to go offline in 2022 will now be operational through at least 2026. The local utility has scrapped plans to install large batteries to store solar power.
Also not reassuringly:
Microsoft was the only one of the four major firms driving the AI boom to answer detailed questions from The Washington Post about their energy needs and plans. Google, Amazon, and Meta offered limited statements.
Carbon zero? More like proof zero
It's extremely tempting to take the tech companies at their word regarding promises of net-zero carbon in the very near future, because we need to drastically reduce carbon emissions if we're going to head off dire environmental catastrophe, and soon. But wanting something to be true doesn't make it true. And meanwhile, the tech companies are non-consensually pushing the output of generative AI on us, whether we ask for it or not. A simple Google search will often now "feature" an "AI Overview" above the other results, and this apparently can't be turned off. Meta replaced their search with "Meta AI". That same "Meta AI" will sometimes jump into discussions in Facebook groups (without even being tagged).
I'm not ready to advise people to stop using search engines or social media. Nor is climate change something which can be solved via individual action. We can, however, be aware of what's happening, and push our elected officials for better climate policy—and know not to fall for Big Tech's promises.