FOMO is not a strategy
End of year reflections on how people are using genAI at work
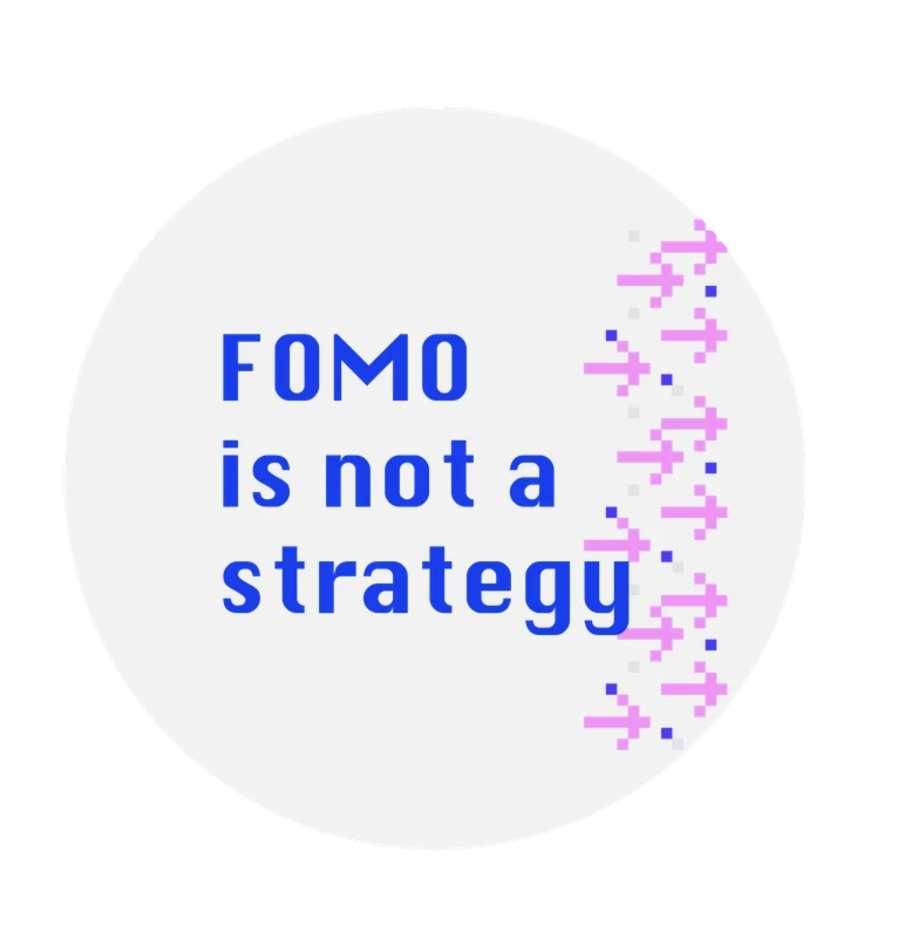
This newsletter isn’t meant to be a definitive “what’s going on with generative AI in 2024”, but a slice of how some people are actually using it.
And I’m going to start with a positive affirmation.
My name is Rachel and I love technology.
The reason I still work in tech after nearly 30 years, and haven’t given it up to open a knitting shop, is because I still find technology to be brilliant and exciting and life-changing. To pick at random: I love using Eat Your Books to search the indexes of every cook book I own, I love carrying my friends and millions of songs in my pocket, and I’m more than a little partial to heartwarming videos about unlikely animal friendships. I love all this stuff - from the trivial and personal to the paradigm-shifting breakthroughs - and it’s an intimate, important part of my life.
And I started with the positive because, honestly, I don’t really like to complain. I like jokes and I’m reasonably good value at parties, but the absolute nonstop cavalcade of Big Tech hype means there is a lot to cut through just now. In particular, there is a barrel-load of shamelessly over-optimistic sales and marketing that is both bending economic policy (such as the Industrial Strategy) and reshaping how government services and workplace IT are delivered. While all eyes might be on Elon Musk’s adventures in the White House, it’s difficult to move in the UK right now without bumping into another massive Microsoft contract or the offer of some gratis Copilot licenses.
In spite of this robust sales approach, it still seems like the economic gains predicted for generative AI are a long way off. Just this week, as ChatGPT celebrated its second birthday, Microsoft published a blog post saying genAI represented a £3.8 Trillion Opportunity for the US economy – but as Parmy Olsen and Carolyn Silverman reported for Bloomberg, the big winners in this economic uplift still seem to be “a handful of tech firms … [that] have seen their market capitalizations grow in aggregate by more than $8bn”.
So, what does this AI transformation actually look like on the ground?
This year, the Careful Industries team has spent a lot of time working with non-technical organisations who are figuring out their approach to AI. What follows won’t betray any trade secrets or client confidentiality, but there are a few things that have surfaced often enough to suggest there are some emerging trends. We’ll probably do a proper corporate write-up of this at some point, and I should probably also sell you our strategic AI services, but – what can I say, I’m very easy to find on the Internet, so let’s do that another time.
For now, I’ll start with three observations.
Super Fans.
There is a small but significant number of people who really like generative AI.
This isn’t the same as the number of people who’ve used (or claim to have used) genAI, but a subset of superfans. I tend to meet two kinds of people in this category: enthusiastic senior leaders who have been tasked with doing something innovative but might not be hands-on users, and more junior people who are enterprising and computer-literate and want to try something new. Notably, some of the people in the second group might have a natural affinity for prompt writing but not enjoy another part of their job – like writing long documents or emails – and so are pleased to outsource those tasks to AI.
Not much system change.
We aren’t encountering many organisations that are doing big, end-to-end overhauls to automate systems at the moment. I’m sure these transformations are happening, but they are expensive and require investment in people and skills, not just one-off capital investments or redirection of a year-end underspend.
Also, if your organisation doesn’t have well-structured data and systems and clearly defined rules and procedures, it’s not possible to just “switch on” an AI project and make savings; more commonly, one discrete part of a complex system is being automated or individuals are left to implement workplace hacks as they see fit - which brings me to:Personal efficiencies rather than organisational gains.
In the workplaces we’ve seen inside, genAI seems to mostly deliver incremental productivity gains for individuals - shaving time off boring or difficult tasks and workflows, or creating a starting point for a creative or administrative task that may otherwise seem daunting. This may be different in e.g. logistics, software and accounting firms where structured data and repeatable tasks are more common, but we’ve not yet spoken to anyone who has (or who is willing to admit they have) revolutionised their entire approach to work so thoroughly as to make themselves redundant.
Overall, the personal adoption of genAI by a small-but-enthusiastic group seems to be the most salient thing for knowledge-intensive business, and it has the following characteristics:
i) The roll-out of genAI tools and gadgets in existing enterprise software and web services means that many people wouldn’t know or notice if they were using AI or not, so self-reporting isn’t very useful.
ii) It is difficult for employers and IT teams to know exactly what tools and software staff are using unless they do a survey or create clear policies – especially if people are moving between the enterprise software and services that come pre-installed on their work computer and doing the odd task in a browser window or on their personal phone or other device. In fact, it’s quite normal for people to have unclear boundaries around using personal devices – for some businesses this is a potential security risk, but it’s also a default part of the modern technology landscape that needs appropriate management. (See, for example, this recent story of WhatsApp use in the NHS.)
iii) Personal workflows are tending to stay localised to one or a small number of people rather than snowballing into wholesale organisational transformation. This makes sense as many desk jobs come with a relatively high degree of worker autonomy and, if there aren’t safety or security reasons to dictate it, no two people will create their spreadsheets or organise their task lists in the same way. This may change over time, but for some people “doing things their own way” and problem solving is one of the things that makes their work interesting and worthwhile.
iv) People who really enjoy using genAI tools seem to particularly like the ad hoc freedom of daisy chaining a few things together and experimenting, and part of the fun is trying things out rather than adopting standardised new protocols. And if every workaround a coworker develops goes on to become standard practice, there are likely to be drawbacks: as well as potentially being irritating for colleagues, there would need to be new routines to manage workflows, such as quality assurance, standards-setting and training.
v) An individual might enjoy using a tool because it gives them an extra 10 minutes here and there, or because it makes a boring task seem more fun, but that does not guarantee their whole working day will become more efficient or productive.
vi) Finally, in most workplaces, incentive structures don’t exist for people to (a) reduce their workloads to such an extent that their role becomes vulnerable or (b) voluntarily accept more responsibility without also taking on more pay.
These things are all natural rate limiters on technology adoption and the precise mix they show up in varies from workplace to workplace as every team has its own culture and ways of working. And regardless of what your friendly neighbourhood management consulting firm will tell you, there’s no one singular set of mitigations to get around this – technology will work best in your workplace if it’s rolled out in tune with existing culture, routines, and ways of working.
Now, of course, these are all observations based on the relatively small set of organisations we have worked with this year and the people we have spoken to, but emerging insights around technology very often begin this way. Usage patterns and terminologies need to be established before better quality data can be generated, and by the time those norms have settled it is likely that a trend will have been baked in. I’m also sure that McKinsey and PwC are currently working hard on very different State of 2024 reports, showing infinite upticks and efficiency gains – and perhaps my scepticism about generative AI means I seek out the flaws, but again, every report you read on this will be biased by either a sales pitch or an ethical position. The Tony Blair Institute can probably show you 100 “number go up” graphs at this very moment, but I tend to think that reality exists in at least several removes from such charts - nestling in the complexity of real-world conditions and motivations rather than the simplicity of an x and y axis.
So, what is the conclusion?
I think, for me, it’s that you can probably go easy on the FOMO.
If your job involves doing something like managing undersea pipelines, predicting bus arrival times or moving lorries with perishable goods across continents, you probably have some pretty good data and a plan for using AI to improve the quality and speed of delivery. If you don’t, now might be a good time to think about how to do that, and how to do it in a way that accords with your organisational values and Net Zero targets.
If your business depends on trust – in delivering services, developing relationships, taking care of people – then generative AI in particular will probably only deliver marginal gains for some individuals, and that may risk the quality of your overall delivery. There might be a good case to empower staff to use genAI and other tools in ways that make their lives easier, but the second- and third-order consequences of those decisions need to be understood if you’re going to carry on delivering business as usual. I’d suggest starting with looking for easy efficiencies that can be delivered without an algorithm rather than trusting in tech to deliver the change. (The Consequence Scanning Tool I co-developed with Sam Brown at Doteveryone is a useful starting point for mapping this.)
Obviously there are lots of use cases for generative AI that sit between these two extremes, but don’t be surprised if the next year sees more individuals craft their own workflows without that turning into particularly notable organisational savings. The sector-changing examples will probably continue to remain edge cases into 2025, relying on disruptive mechanisms rather than incremental change, and I’m pretty sure you can hold your nerve before Year End without buying any more Copilot licenses.
Nothing about AI is inevitable — and generative AI is just a series of tools. If the person who created the spanner was insistent that spanners had to be attached to everything, life would have gotten boring pretty quickly.
Remember, FOMO is not a strategy – or at any rate, it’s never a good one.