Well, that can’t be right…right?
I can’t help it. Suspect data jumps out at me.
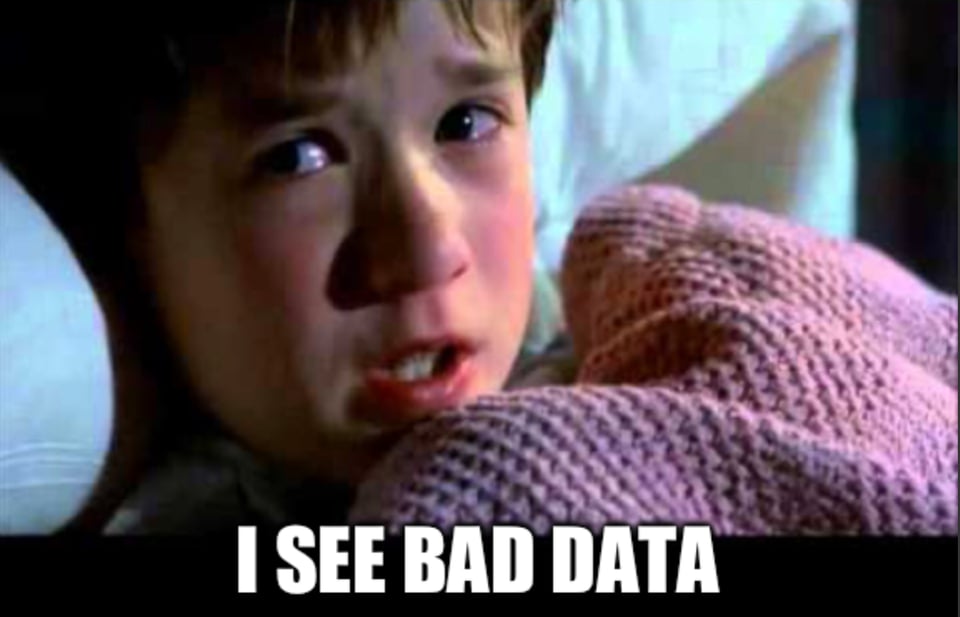
I logged into my utility provider's website to pay my gas bill earlier. I'd forgotten how much higher those things get around here in the winter, and decided to take a closer look at projecting how much I should be expecting to pay over the next few months.
Like many utilities, my natural gas provider includes a couple of handy charts and tables on their bills and website to let me know what my usage trends look like over time. I look at these each month — they’re handy cues for knowing when it’s time for a showdown over the thermostat settings or when to start yelling at people for taking too long in the shower. Historically, I tend to pay less attention to the local temperature data they also include, but I'm paying more attention to outdoor temperature extremes heading into this winter than I've ever been before, because now I’m obsessing about the survival chances of my honeybees. So, this time, a little anomaly jumped out at me:

Whaaaat? The highest temperature in the entirety of last January was 25 degrees Fahrenheit? A full 38 degrees lower than the high for any other month? I had a visceral reaction…That can’t be right. My spidey sense data quality radar tingled. I could, of course, find out whether I was right with a simple Google query — historical weather data is, after all, pretty easy to track down. But since I’ve been having a lot of conversations lately about how to help others develop analytical thinking skills in general, and data quality management skills in particular, I thought this might be a good opportunity to take the scenic route.
The first thing I do when I set out to triage a suspected data quality issue is ask myself a few initial, grounding questions:
What kind of possible data quality problem does this represent?
How important is this, really, and what are the potential consequences of ignoring this type of problem?
What other data could I use to help me determine whether there is an issue of this sort in my dataset?
Is that other data easily/inexpensively/reliably available?
Who (besides me) might care about issues of this sort?
And, very much not least of all:
- What biases have I brought and what assumptions have I made that may be setting me up to be totally wrong about this?
In my next post, I’ll dig into the motivation for these questions, work through answering them, and set about making a determination of whether or not the data, or my knee-jerk reaction, is to be trusted...this time.