The absurd amount of energy LLMs use
Let's say you want to use the full DeepSeek-R1 LLM on your machine. The full model uses about 1300 GB of RAM on the GPU for any reasonable performance. Nvidia's most expensive desktop GPU, the newly released GeForce RTX 5090, has 32 GB of RAM, costs 2000 USD, uses 575 watts, and you need a computer around that GPU. The rest of the computer, CPU included, uses about 200 watts.
For some scale: My laptop uses 130 watts on full power and 10 on idle. An electrical heater uses about 800 watts A Fiat 500, a compact car, has 70 hp. Yes, hp as in horsepower. 1 hp is 745 watts. Your entire system uses 1 horsepower and is completely unsuitable for this task.
If you think 2000 USD is a lot of money, do not worry. NVIDIA sells GPUs with more RAM for data centers which cost way more than the consumer GPUs. NVIDIA has some competition on gaming GPUs, but none whatsoever in deep learning.
You can get the GPU model DeepSeek reportedly used, the H800, in neat packs of 8 in a box ready to insert in a rack. Each pack costs around 300k USD in 2025, with hopelessly outdated GPUs. You will need two (maybe three) of those boxes interconnected and set up properly just to use the LLM. The boxes alone use 8hp, not considering the network equipment and air conditioning. After all this trouble, and you get… some words per second. There are people, right now, bragging they made a lighter version of DeepSeek-R1 run at 40 words per second on a cloud cluster. At a substantial amount of the power required to drive a car.
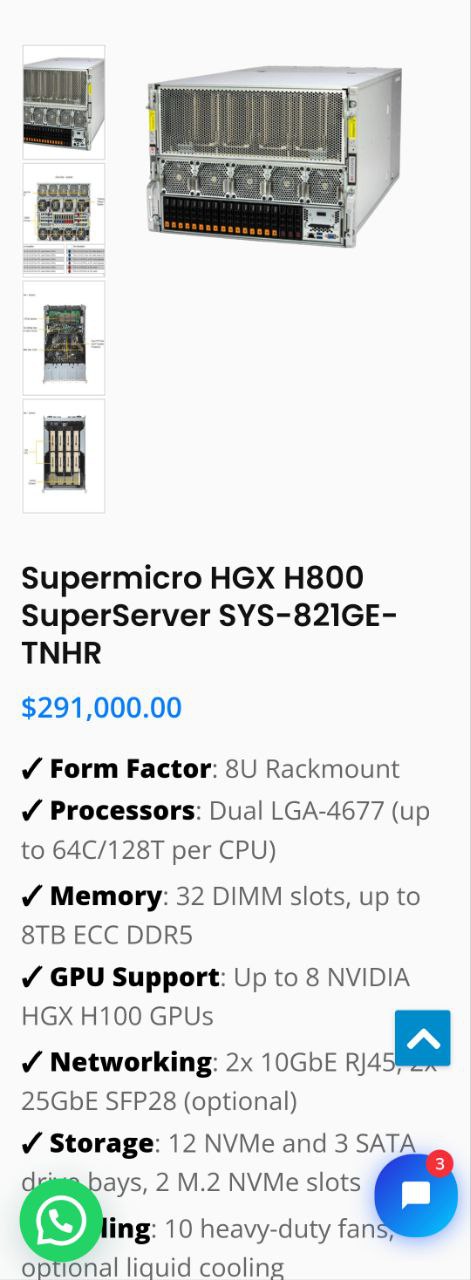
For that amount of money, on normal web applications, you can have tens of thousands of users at the same time without breaking a sweat. Depending on the application, hundreds of thousands or even millions of users. I have been in that position, and my team definitely didn’t spend millions on compute — caches are a wonderful thing.
Remember, a big part of the noise is about how this LLM is far more efficient than the competition.
Some caveats apply, but they don't change much of the equation. Let's talk about them:
Distillations
Long story short, distillations are smaller versions of the main LLM that retain most of the quality while using fewer resources. Another reason for the general panic is that R1's smaller versions retain more quality than the competition. One of the smaller versions uses… about 800 GB of RAM, so you’d still need one of the crazy expensive machines at least. There are smaller versions, yes, but the ones that can run on “normal” computers aren’t that great (I tried).
Inference Chips
Reportedly, the inference for DeepSeek is happening not on NVIDIA chips, but on local chips made by Huawei. Because of the US embargo, they aren't as efficient as anything NVIDIA makes, but they are certainly cheaper than NVIDIA's — and they aren't embargoed.