Technique, tranferable skills and new paths
Hello!
You are reading (Dataviz) Design Matters - a newsletter series made with 💕 by T. from Data Rocks.
If you’re new here but like what you see, subscribe below and get all upcoming issues straight into your inbox.
Tools go, technique stays
As someone who’s transitioned from business roles into data roles, I am often asked what advice I’d give to someone just starting out - either as a fresh grad going to data roles or as a seasoned professional that, by choice or accident, has landed a data role. I suggest this: focus on the technique before the tools.
I don’t mean that you don’t need tools at all - of course, you do. But tools, especially when we’re talking about technology, come and go. They can quickly become obsolete, outdated, or stop being relevant. But if you understand the more essential, structural bits of what makes a data visualisation good, you’ll be able to produce good results with whatever tools may be at hand.
Very much like an artist holding a chisel, if you have an understanding and a clear vision of what you want a piece of marble to be, you’ll employ your tool with the proper techniques until you get to your desired result. If you have a hammer instead of a chisel, the same vision and techniques will still apply, even if using the hammer may be more difficult at first. You’ll soon get the hang of it and achieve a similar result.
It may seem all too overwhelming because so much focus is given to the technology - but if I could start learning data visualisation again, this is what I’d do:
- I would pick one tool only and be sufficiently good at it - no need to learn all the ins and outs of every feature. Learn enough to get the job done;
- Then, I’d focus the rest of the time learning about what makes a visualisation truly good. What sets an outstanding piece of work apart from the rest? What techniques were employed? How can I make it happen with the knowledge I have right now in my tool of choice?
- I’d go from there - I’ll inevitably want to learn more about how to make cool stuff happen in my tool of choice. Before I know it, I’ll have learned way more than if I tried to take a traditional approach instead. And what’s best: I will have a bunch of cool examples to derive other good things from.
Considering this approach, this past 4 weeks, I have reviewed a few books that can help guide you if you’re starting out. First, I talked about the non-glamorous path to becoming good with data, taking the 7-step process for analysing data from the book The Accidental Analyst. Then, I made a case for asking good questions and why they’re essential for any good analysis. Next, we went a bit further up the chain and learned about what a good data strategy looks like and why leadership buy-in is paramount to ensuring data initiatives are successful. And last week, I talked about the importance of data communities.
None of these articles focused on a particular tool. I believe a data culture can be successful regardless of which technology they choose to adopt. Leadership, analytical skills, curiosity, a desire to learn and solve problems, and strong community-building around data themes are much better enablers of success than any tool-focused training.
The part where I talk about A.I.
Everyone is taking their bets and making their reckons, so I shall make mine as well. The recent developments in A.I. are exciting. ChatGPT is sweeping the world, and I can’t scroll anything online without seeing at least a few mentions about it.
I must confess I am not particularly concerned with it taking my job. At least not until it takes a few further considerable leaps. It’s anyone’s guess how long that may take, but I am reasonably confident that whatever may happen next, human supervision will still be required.
The work I do is highly personalised. Most of the time I spend on a project is translating requirements into a form I can work into some sort of visual representation. The client often doesn’t know how to achieve their vision by themselves, so they need someone else to help them. The main advantage I have over an A.I. chatbot is contextual: I can sit with my client and bounce back ideas until I fully comprehend what they mean with their requirements before trying to create anything. I understand there will always be a gap between what the client asks for initially and what they intend to achieve. An A.I. chatbot doesn’t acknowledge that; it takes requirements at face value. It spits out a result based on a prompt - without necessarily working out if the prompt makes sense in the user’s context.
It also means that, although an A.I. may be capable of replacing currently existing tools that I use to create data visualisations, it won’t immediately be able to create particularly useful, curated and ideal visualisations considering the context in which these creations will exist. Even if I incorporate A.I. as a tool to help me achieve an end, the human counterpart bridging the gap between the user and the technology will still be necessary. I’ll still have a job, even if different in nature.
Another point that has been brought up multiple times is that of legislation around A.I. content. Who owns it? Should articles and images written by A.I. be allowed by publications? Should we work out ways to identify content created by A.I. and label it as such? The discussions are just in their infancy, and we’ll surely hear a lot about it.
In the meantime, you may have noticed I have added little badges to my articles, saying they’re all created by a human (me!), not an A.I. They come from the Not by A.I. project, which I first read about in Duncan Geere’s newsletter.
On this note, I found a few interesting points and discussions around the topic of A.I. recently.
- First, this piece discussing the almost cult-like language we hear around A.I. and technological transformation.
- Second, Ben Jones’ view on the letter circulated last week asking for all development of A.I. technologies to halt until regulations are in place. He summarised the same thoughts I have about the subject, only much more eloquently than I ever could. It is worth a read.
- Last, Nick Desbarats’ article wondering if A.I. will automate Data Visualisation.
Other interesting bits and bobs:
-
I came across this very well-written piece about inclusivity and technology, where they argue about the inherent bias that comes with using predominantly W.E.I.R.D. populations as the point of reference. That is: Western, Educated, and from Industrialized, Rich, and Democratic countries.
-
And to end on a delightful note, illustrator Lian Cho will teach you how to draw 100 dragons.
Last but definitely not least, I am looking for my next awesome client! My schedule will be available starting May 2023. If you have a cool project involving data visualisation, reach out either through this email or my website and let’s chat!
If you’d like to keep the conversation going, just reply to this e-mail. I’d love to hear your thoughts!
See you again in 4 weeks!
-- T.
If you’d like to keep the conversation going, just reply to this e-mail.
This newsletter is a labour of love, researched, written and designed fully by me, alone.
A lot of effort goes into it, so every bit of help counts!
Here’s how you can support the (Dataviz) Design Matters newsletter:
Tell your friends! You can forward this email to them, or ask them to subscribe using this link.
You can buy me a coffee.
Or you can become a V.I.P. (which stands for Very Interesting Pie) subscriber and get new articles a month before everyone else, as well as my unending gratitude.
And here’s how else I can help you:
You can get helpful resources at the Data Rocks shop.
You can book a Rescue Session with me.
You can check out one of my Mini-Workshops.
Or get in touch?subject=Project Inquiry) to discuss that cool dataviz project you might need help with.
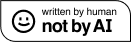
If you want to use any of my writing or materials in your work, please do so with attribution. Copyright © Data Rocks 2019-2024.