Design Matters, and can ChatGPT help me with colours?
Hello!
You are reading (Dataviz) Design Matters - a newsletter series made with 💕 by T. from Data Rocks.
If you’re new here but like what you see, subscribe below and get all upcoming issues straight into your inbox.
Buckle up! This one's gonna be long!
I took a small break from client work these past four weeks. As much as I love seeing a piece of work delivered to a client and watching as they discover new ways to weave data into their day-to-day processes, I enjoy dedicating a bit of time to other sides of data viz - beyond the dashboard crafting that I normally do.
So, I had more time to dedicate to side projects and try new things.
I have played around quite a bit with ChatGPT with the main side project I'm working on - particularly to help me establish an easier way to craft colour palettes that can be used for dashboard design.
In this issue of What's Rocking, I'll share a bit about this side project and what it's like to ask a chatbot with no eyes or visual perception to help you create something involving colours.
You may notice that I wrote articles about only three books this month instead of four. I needed to re-focus my time and shuffle things around a bit, so instead of publishing one new article every week, they'll come every other week. Next month, I'll post two, and I intend to keep that schedule for a while.
Publishing every week is awesome for engagement, but I like quality and long-form writing - so I'd rather do less of it and keep them at the same level.
From the Data Viz Bookshelf:
- I began the month with Dear Data and Observe, Collect, Draw - two awesome books by Giorgia Lupi and Stefanie Posavec, which talk about their journey with physical data visualisation - using paper and lots of colourful pens.
- Then, I shared one of my all-time favourites for anyone serious about getting into datavis as a career. Tamara Munzner's Visualisation Analysis & Design, THE classic textbook I always go back to in my shelf - my copy has a trillion little post-it notes hanging from its pages.
- And finally, I talked about Data Sketches by Shirley Wu and Nadieh Bremer. This book is not only gorgeous, but it takes the reader through their journey and process of crafting 24 amazing visuals across 12 different themes. The level of detail and care is off the charts! (pun intended).
Design matters:
When I first started creating data visualisations, it was as an analyst in need of communicating complex data and concepts quickly. Many in the field share this origin story: tasked with making vast amounts of information accessible to those with limited time and patience to sift through countless rows and columns in search of crucial data points.
This means that I learned datavis from a purely utilitarian standpoint: data visualisation is there to be strictly functional. Many analysts share this view, which can lead to an unintended consequence: anything beyond minimum utility is considered an unnecessary cost.
Debating design's role in data visualisation can be tough. Those with technical backgrounds and a utilitarian perspective often argue that as long as there's function, form isn't necessary.
Tool vendors' tendency to downplay the effort behind high-quality data visualisation doesn't help either. They sell it as simple as drag-and-drop, with the tool doing everything automatically - but the reality is far from that.
This mindset fails to recognise that design encompasses both function and form, with form enhancing function.
Data & Analytics teams often express concern about the low adoption of their diligently developed reports and dashboards. Despite having accurate and complete data, users still gravitate towards using spreadsheets instead of the solutions they developed. It is a frustrating experience for everyone involved: so why does it keep happening?
The answer can usually be summarised as "design". Or better: the lack of it.
By design here, I don't mean the looks. It refers to the systematic organisation of visual elements that guide users through clutter, enabling them to locate information efficiently while minimising cognitive strain.
It is the art and science of using a digital interface to guide and enable, to make things more accessible to a wider audience. This is what design does. In particular, what UI (User Interface) Design and UX (User Experience) Design do.
Adopting design systems and processes in Data & Analytics projects inevitably results in better user adoption.
The caveat is that good design is an expertise of its own. Designing a good user interface involves a lot of microdecisions. A Data Analyst is probably already overwhelmed by their job's demands and by constantly having to be at the forefront of an environment of ever-shifting technologies.
So here I come, telling them that, beyond knowing all there is to know about data, they also need to learn about stuff like choosing colours, contrast ratios, harmonic scales of typography, what a drop shadow actually does, where to place stuff on a screen, visual hierarchy, etc.
But if you want to take your dashboards from just enough to awesome, you'll have to invest in design expertise. Be it by helping your analysts to embed design as part of their development process or by hiring someone with this expertise who can collaborate with them and help lift their design practice.
If all of this sounds a bit daunting, don't worry! I'll start sharing more about what this looks like in my newsletters beginning today.
The multiple visual functions of colour in data visualisation
When it comes to dashboards, one of the main pieces that usually has significant room for improvement is the use of colours.
In my experience, the main struggle comes from trying to use colour as both the visual identity of a brand and as data encoding.
If you look up colours for data visualisation online, you'll find heaps of resources discussing the usage of colour to represent data - as a category or scale. In other words, we know about the use of colour to encode data, but it is rare to see the mention of other functions that colour can have on a user interface.
Should you colour the background? What colour should your text be? Should different types of text be of different colours? What about alerts? Information boxes? Icons? Do all of these need to also be brand colours for my dashboard to be considered "on brand"?
Colour is often over-utilised as encoding, too: often, the same story or message can be communicated using other encodings, and yet, we end up with stacked bars with legends including 20 shades of blue.
And I haven't even brought up the matters of accessibility! Not just colour blindness but contrast. If two colours ate too similar or if a text is too light against a light background, many of us will struggle.
A lot of thought goes into deciding on a proper colour palette. If you're under the pump to deliver a dashboard in record time to an anxious group of stakeholders, you'll likely just go with tool defaults. And if there's one truth about datavis tools, it is that tool defaults are rarely ideal.
Can ChatGPT help?
This is a question I'm getting more and more whenever I raise a point like this, no matter how trivial or big - can't ChatGPT just do it for you?
So, as the starting point of my side-project (wink, wink), and also in the spirit of trying to make it easier for all of us busy people, I set out to ask ChatGPT to create the perfect colour palette for a business dashboard.
A few disclaimers:
- I am using Chat-GPT4.
- I don't have access to any of the plugins yet (I'm on the waitlist, though).
- The example images are made using Figma to help me evaluate what the palettes look like in context.
Prompt 1:
Could I just ask ChatGPT, straight up, to create a colour palette for a dashboard? Let's see:
Some observations:
- Interestingly, it went with a dark mode dashboard. I wonder where it took that from!
- The off-white text against the dark blue background is an AAA score for contrast - very good!
- As incredible as it may sound, there seems to be good contrast between the green and the yellow when compared to the dark blue background too!
- The problem comes when comparing the encoding colours against the background - they've consistently failed contrast tests.
- It is also quite curious that ChatGPT mentions these colours specifically for chart elements, considering them as highlights (yellow, red, and blue).
- The functions of the colours are a bit unclear, though. What does it mean by alternative background? I used it as the sidebar colour, but that's unclear.
- The same goes for all the mentions of text colours in different hues. What's up with that, I wonder?
- I reckon I wasn't expecting the blurb about accessibility. Nice touch, bot.
- All in all, it is technically good, but it doesn't look polished, professional or modern. It is quite heavy, and I must say hard to distinguish what's important from all the noise the dark colours create.
Prompt 2:
Ok, that was a somewhat promising start, even with some hiccups.
But what would happen if I offered it a brand colour to take as a reference?
Let's imagine we're creating a colour palette for a company that wants their dashboards to be on-brand. Let's imagine this is for a beverage brand that has a red and white logo.
- Overall, the result looks like it was meant for a fast-food company instead of a beverage one.
- Also, this was me being a bit mean to the bot. Red, yellow and green are notoriously difficult colours to use as primary references for any dashboard. What should the alerts look like if everything is red and yellow? Tough question.
- Speaking of red, yellow and green - have you noticed that it wants to include these three hues in any prompt's results? My hunch is that most dashboards have some type of semantic colour palette (the alerts or KPI colours), and it's feeding from that.
- Even though the text greys pass accessibility tests based on the background option - light grey and white - You can't use any of the other colours with the text options. (see the button, for example).
- Again, there's no nuance - all colours have high saturation, and all of them are quite solid.
- Colour can also be used to reinforce visual hierarchy, and when all of them look just as strong, that becomes more difficult to do.
Let's think about it a bit before more prompts.
When I and my human eyes and my human perception of colour create a colour palette for a dashboard, I take a few important things into consideration.
Here's the list of colour functions I use and how I decide what each of them looks like.
There are three main groups of colours that make a good dashboard. And I usually follow a common UI design rule called the 60%-30%-10% rule. This means that 60% of what's on an interface should be made of neutral colours, 30% should be your main colours (brand identity, your data colours), and 10% are accent colours (they call attention to certain details - usually your alert colours).
With this in mind, this is what I plan to have:
- scaffolding colours (axis, titles, subtitles, strokes, icons, annotation text) - neutrals - 60%
- encoding colours (data colours, categorical, diverging or sequential)- primary colours - 30%
- semantic colours (usually some version of green-yellow-red) - alerts, KPIs, small details - 10%
Each one of these groups is composed of a small range of colours. I could go on about the criteria and colour theory to choose each tidbit of them forever, but this is a newsletter, not an essay. So let me just say that this is the list I usually go for:
Scaffolding:
Background colours:
- Background surface, light
- Background surface, dark
- Text, light
- Text, medium
- Text, dark
Chart elements colours (axis, labels, titles, annotations):
- Text, dark
- Text, medium
- Text, light
All of the text and surface colours must have a contrast rate of AAA.
UI elements (buttons, bars, icons, etc.)
- UI Surface, Light
- UI Surface, Dark
- UI Surface, Primary (great for buttons)
- Text, dark
- Text, light
UI Surface colours must have good contrast against background surface colours, and all text colours should have good contrast against their surfaces (e.g., text, dark should score AAA in contrast against Surface, light).
Encoding colours
- Up to 3 main colours I'll use to encode data. They should have some sort of rules to keep them visually consistent - like being complementary or analogous colours.
- Each one of them must have the following variations:
- Text, dark
- Surface, Light
- Base colour
- A light tint
- A dark shade
- 3 times 5 = you'll have 15 encoding colours to play with.
Semantic colours:
- One positive colour - normally green.
- One Waring colour - normally orange or yellow
- One Negative colour - normally red
- One information colour - normally blue
Each one of these has three variations:
- Text, dark
- Surface, Light
- Base colour
- For good measure, always have a white colour and an off-black colour.
- Optional: If you want to be extra, throw in a grey scale (up to 10).
- If the brand colour is too bright, it is possible to adjust it a bit and still keep an overall identity. Alternatively, it can be used for small accents instead of the main encodings while keeping most of the interface in shades of grey and neutral tones.
This is what a full-colour system like this looks like, as a reference:
It becomes less scary when we see it, right?
Can ChatGPT do it, with all of this taken into account?
Amazingly, yes. It took me some good two weeks tweaking the prompt, but we eventually got there.
Things I learned:
- be very specific. Vague instructions lead to bad outputs.
- reduce as much ambiguity from the request as you possibly can. The clearer you are, the more detailed you can be, the better the output.
- repeat instructions that apply to specific parts in the beginning, the end, and every time it needs to apply them. You'll notice my prompt is huge mostly because I repeat the same set of commands over and over for each section of colours. It works. If you remove the repetition, you introduce ambiguity, and the bot gets confused.
- give it a role. Tell ChatGPT who they should be acting as. It is incredible how much it helps in the quality of the output.
- it can't see colour, but it knows advanced colour theory and deals well with jargon - it thrives with overly technical instructions.
- ChatGPT likes instructions organised in markdown for longer prompts. You'll notice my prompt is formatted, and it has a few symbols indicating headers, subheadings and bullet points. ChatGPT actually understand how to interpret them and yields better results if you organise prompts like this!
- It won't get it right with all colours you give it as a reference - it does really well if you start with a good set of three colours as a reference, but if they're either red, yellow, or green, you'll get funky results.
- You'll still need to make some adjustments to the brightness or saturation levels of some colours to achieve optimal accessibility, but it does a good job most of the time. I'd say it creates good combinations out of the bat about 7 out of 10 times.
So, in the end, this is what it generated for me. I used three quite challenging colours as a reference on purpose to see what the end result would look like.
It turned out to be pretty good if you ask me.
And here's the prompt, in case you want to use it and have a play with it yourself!
Replace the colours in the reference colours section of the prompt, and have fun.
Let me know if this works well for you too!
IMPORTANT UPDATE!
- As of 12th May and with the addition of plugins, this prompt seems to have stopped working for now.
- I tried using it and adjusting it a few times ever since, and Chat-GPT4 throws a blurb about how it is a language model and it can’t generate HEX codes or work with colour theory.
- I’ll keep trying to debug, and I’ll leave the prompt here for future reference. After all, it has worked at some point, and it is kind of history now.
- If you have any ideas in the meantime, let me know!
A system to help with microdecisions
In 2019 I started learning a design tool called Figma. Figma is a tool for creating user interfaces, and user experience flows, akin to Adobe XD and Sketch. It is heavily used by the web design community.
Back then, I started to organise a set of components, layouts and backgrounds I often use when creating data visualisations. With time, this evolved into a system. I now use it to inform, categorise and document all those design microdecisions I repeatedly make when starting a new project.
It is now a Data Visualisation Design System, and it helps the analysts I work with to become better at design.
This system helps me:
- Prototype and mock-up a possible dashboard's interface before I have the data ready to be consumed by tools like Tableau and Power BI;
- Discuss a vision of the project with clients and stakeholders, helping to show them what can be achieved before spending countless hours in development;
- Keep interfaces and design decisions consistent when working with a bigger team - since it's all documented and easily accessible;
- Keep all design decisions, guidelines and documentation (like colour palettes, font sizes, layouts, and templates) organised in one big repository;
- Keep track of design iterations and modifications;
- Create backgrounds, sidebars, top bars, buttons, icons and other visual elements that can help enhance the overall dashboard experience but are not natively available in BI tools.
Now, to the announcement:
I am working on converting this personal Data Visualisation Design System into a digital product!
Right now, I am about 60% of the way in, and I intend to share some sneak peeks with you soon.
If this is something that may interest you, reply to this e-mail and let me know what you think! If you have questions, ask away!
See you again in 4 weeks!
-- T.
If you’d like to keep the conversation going, just reply to this e-mail.
This newsletter is a labour of love, researched, written and designed fully by me, alone.
A lot of effort goes into it, so every bit of help counts!
Here’s how you can support the (Dataviz) Design Matters newsletter:
Tell your friends! You can forward this email to them, or ask them to subscribe using this link.
You can buy me a coffee.
Or you can become a V.I.P. (which stands for Very Interesting Pie) subscriber and get new articles a month before everyone else, as well as my unending gratitude.
And here’s how else I can help you:
You can get helpful resources at the Data Rocks shop.
You can book a Rescue Session with me.
You can check out one of my Mini-Workshops.
Or get in touch?subject=Project Inquiry) to discuss that cool dataviz project you might need help with.
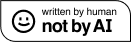
If you want to use any of my writing or materials in your work, please do so with attribution. Copyright © Data Rocks 2019-2024.