An explanation of p-values, effect sizes and more
1 - p-values, misconceptions and different approaches
There are a lot of critics on how scientists have been using p-values. In the blog post below they discuss more about the definitions of p-values and possible alternatives to its usage.
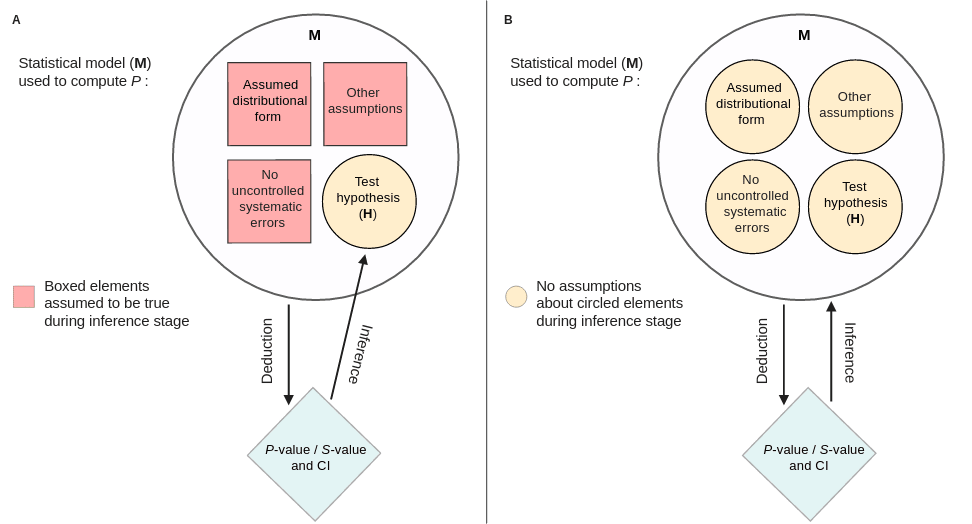
P-values Are Tough And S-values Can Help | Less Likely
An extensive discussion about what P-values are, their properties, common interpretations, misinterpretations, and how a measure called an S-value may better help us interpret them.
2 - Effect sizes or p-values?
On the other hand, several other people claim that p-values receive too much attention and effect sizes are left behind. Below the author discusses more about the implications of using p-values without considering the effect sizes.
Effect size is significantly more important than statistical significance. – arg min blog
Musings on systems, information, learning, and optimization.
3 - Tuning parameters in small and sparse datasets. How good is it?
In the paper below the authors study the effects of ridge regression and its parameters complexities in small and sparse datasets. They describe possible problems and in the end they provide some recommendations on how to deal with these cases. One point worth noting is the specification of priors to the parameters to stabilize the estimates.
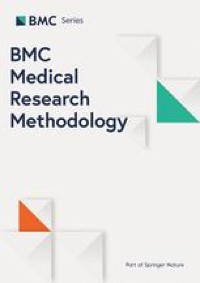
To tune or not to tune, a case study of ridge logistic regression in small or sparse datasets | BMC Medical Research Methodology | Full Text
Background For finite samples with binary outcomes penalized logistic regression such as ridge logistic regression has the potential of achieving smaller mean squared errors (MSE) of coefficients and predictions than maximum likelihood estimation. There is evidence, however, that ridge logistic regression can result in highly variable calibration slopes in small or sparse data situations. Methods In this paper, we elaborate this issue further by performing a comprehensive simulation study, investigating the performance of ridge logistic regression in terms of coefficients and predictions and comparing it to Firth’s correction that has been shown to perform well in low-dimensional settings. In addition to tuned ridge regression where the penalty strength is estimated from the data by minimizing some measure of the out-of-sample prediction error or information criterion, we also considered ridge regression with pre-specified degree of shrinkage. We included ‘oracle’ models in the simulation study in which the complexity parameter was chosen based on the true event probabilities (prediction oracle) or regression coefficients (explanation oracle) to demonstrate the capability of ridge regression if truth was known. Results Performance of ridge regression strongly depends on the choice of complexity parameter. As shown in our simulation and illustrated by a data example, values optimized in small or sparse datasets are negatively correlated with optimal values and suffer from substantial variability which translates into large MSE of coefficients and large variability of calibration slopes. In contrast, in our simulations pre-specifying the degree of shrinkage prior to fitting led to accurate coefficients and predictions even in non-ideal settings such as encountered in the context of rare outcomes or sparse predictors. Conclusions Applying tuned ridge regression in small or sparse datasets is problematic as it results in unstable coefficients and predictions. In contrast, determining the degree of shrinkage according to some meaningful prior assumptions about true effects has the potential to reduce bias and stabilize the estimates.
4 - To umap or not to umap?
Recently several researchers have been discussing the validity of umap in single cell RNA-seq datasets. In the following papers and threads, researchers debate some technical issues and whether one should use or not umap.
The links below should be read in sequence, as they represent the flow of the debate.
It's time to stop making t-SNE & UMAP plots. In a new preprint w/ Tara Chari we show that while they display some correlation with the underlying high-dimension data, they don't preserve local or global structure & are misleading. They're also arbitrary.🧵https://t.co/XkAOTKlOcs pic.twitter.com/dmFzD5RR6R
— Lior Pachter (@lpachter) August 27, 2021
I am late to the party (was on holidays), but have now read @lpachter's "Specious Art" paper as well as ~300 quote tweets/threads, played with the code, and can add my two cents.
— Dmitry Kobak (@hippopedoid) September 13, 2021
Spoiler: I disagree with their conclusions. Some claims re t-SNE/UMAP are misleading. Thread. 🐘 https://t.co/yFLgz3bo6X pic.twitter.com/Ky9QRmXoyS
In response to questions & comments by @hippopedoid, @adamgayoso, @akshaykagrawal et al. on "The Specious Art of Single-Cell Genomics", Tara Chari & I have posted an update with some new results. Tl;dr: definitely time to stop making t-SNE & UMAP plots.🧵https://t.co/c1yIWm4BLz
— Lior Pachter (@lpachter) September 22, 2021
Chari et al. (@lpachter) have updated their preprint and doubled down on their claim that an 🐘-looking embedding, a random (!) embedding, and 2D PCA, all preserve data structure "similar or better" than t-SNE.
— Dmitry Kobak (@hippopedoid) September 23, 2021
I still think this claim is absurd. [1/n] https://t.co/f90LAdLIIB
5 - Integrating Clinical Omics in Precision Management of Breast Cancer
This week there will be a virtual meeting discussing the current and future challenges of using omics datasets for biomarker and clinical diagnostic assays development in breast cancer. The registration fee to participate in both days is 120 pounds.
https://www.royalmarsden.nhs.uk/clinical-omics-technologies-precision-management-cancer