AI Week Jan 22nd: AGI ambitions, poisoned AIs and comics
In this week's AI Week:
- ChatGPT's take on science illustration
- What's next for AI? (AGI / LBMs / LAMs)
- Comics Section: Penny Arcade and SMBC take on AI
- Headlines (Poisoned LLMs / ChatDPD / Bad translations / Geometry proofs)
- Longread: 4000 of my closest friends
This week's AI-generated image:
Source: https://twitter.com/JeffYoshimi/status/1748086115498897469
Science illustrators, your jobs are safe.
What's next for AI?
In the last week, I've noticed three things jostling for the space of "the next hot thing." 2023 was very exciting for LLMs and image generation. Now, a bunch of companies want you to know that the thing they're working on will be the LLM of 2024, or maybe 2025.
First, two things: 1) There are a lot of candidates for this slot; these are just the three that jumped to my attention this week. And 2) We haven't solved LLMs and image generation. If you've been reading my newsletter for a bit (and if you haven't, welcome!), you've seen me mention some of the major issues like "hallucination" (LLMs making stuff up), "toxic content" (generating bad stuff), and plagiarism/IP abuse. I haven't gotten into all of the issues -- generative AI also has a racism problem, and image generation struggles with text, for example.
Anyway, the three things that stood out to me this week were AI-powered robotics, Artificial General Intelligence (AGI), and AI agents.
AGI?
Meta announced this week that they're going to buy more Nvidia AI chips than anyone and build artificial general intelligence (AGI).
"It’s become clearer that the next generation of services requires building full general intelligence." -Zuck
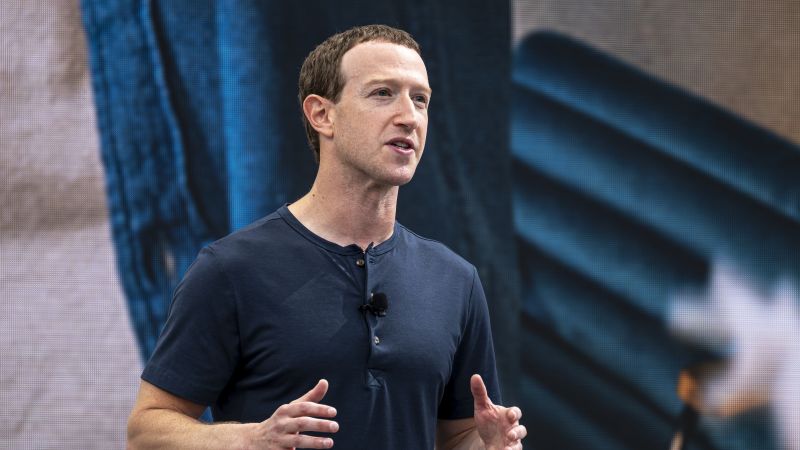
Meta CEO Mark Zuckerberg makes biggest pledge to AI yet | CNN Business
Meta is getting more serious about its place in the growing AI arms race.
And Sam Altman, CEO of ChatGPT maker OpenAI, said that "human-level AI" is coming soon but won't be a big deal. This is the same Sam Altman who warned the US Senate last May about the potential dangers posed by powerful generative AI models. "I do think some regulation would be quite wise on this topic," Altman said at the time.
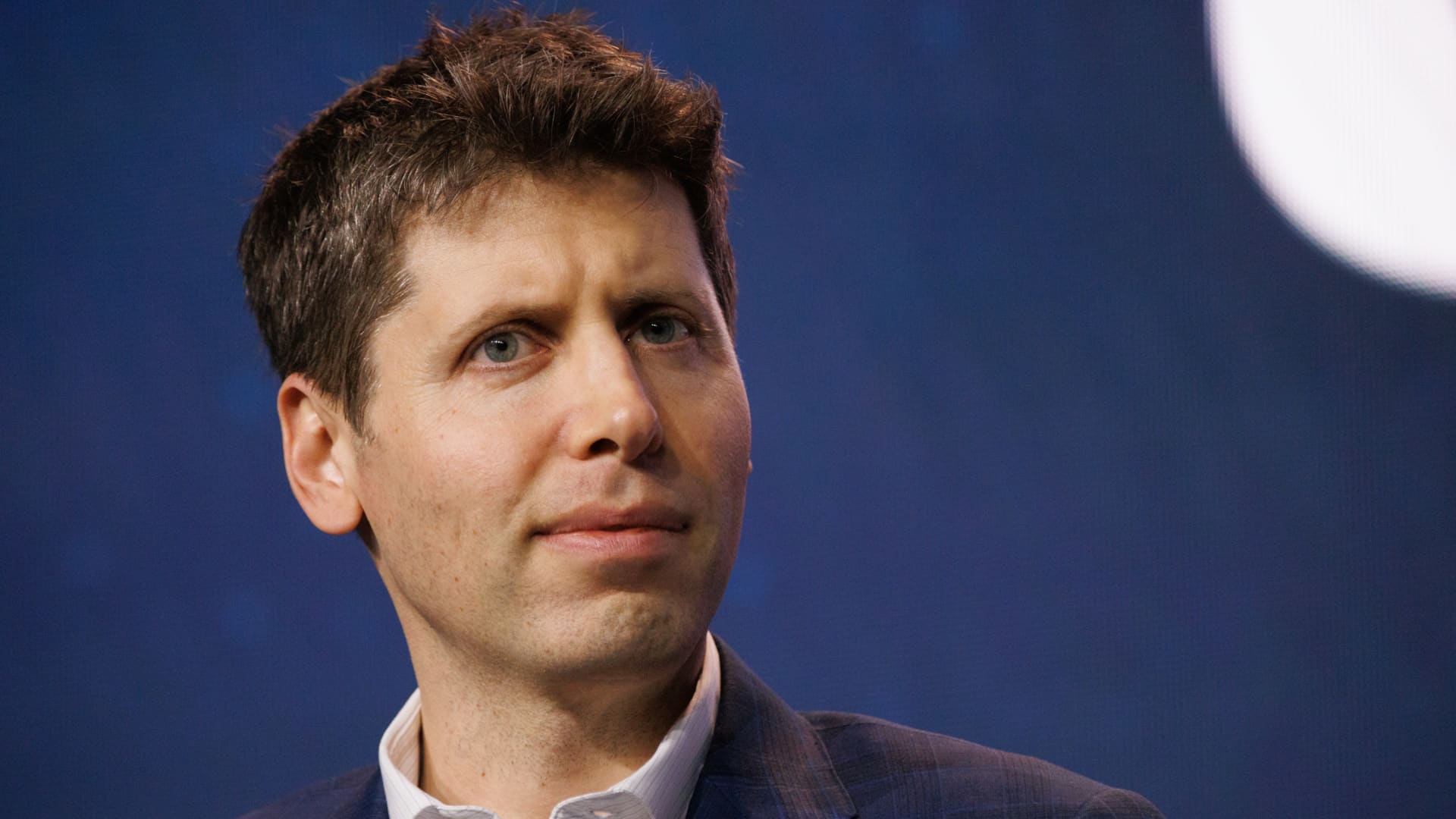
OpenAI's Sam Altman: AGI coming but is less impactful than we think
Sam Altman said concerns that artificial intelligence will one day become so powerful that it dramatically reshapes and disrupts the world are overblown.
LBMs (no relation to LGM 👽) for AI-powered robots
Who's living the Jetsons dream with a robot maid? Not Elon Musk! Last week, he tweeted (Xeeted? -- ugh, sorry, I still can't) a video of Tesla's humanoid robot, Optimus, folding a shirt. Wooo Jetsons Dream!
Important note: Optimus cannot yet do this autonomously, but certainly will be able to do this fully autonomously and in an arbitrary environment (won’t require a fixed table with box that has only one shirt)
— Elon Musk (@elonmusk) January 15, 2024
Except... Optimus wasn't folding the shirt by itself. Just visible in the lower right corner: A telepresence glove making the exact same moves. Busted!
'Hand' in lower right at edge of screen comes in/out of frame echoing movements. 👀👀👀. . pic.twitter.com/rW5AjHY2nW
— Lisa #TeslaTruth (@TeslaLisa) January 15, 2024
Yes, a very important note, indeed.
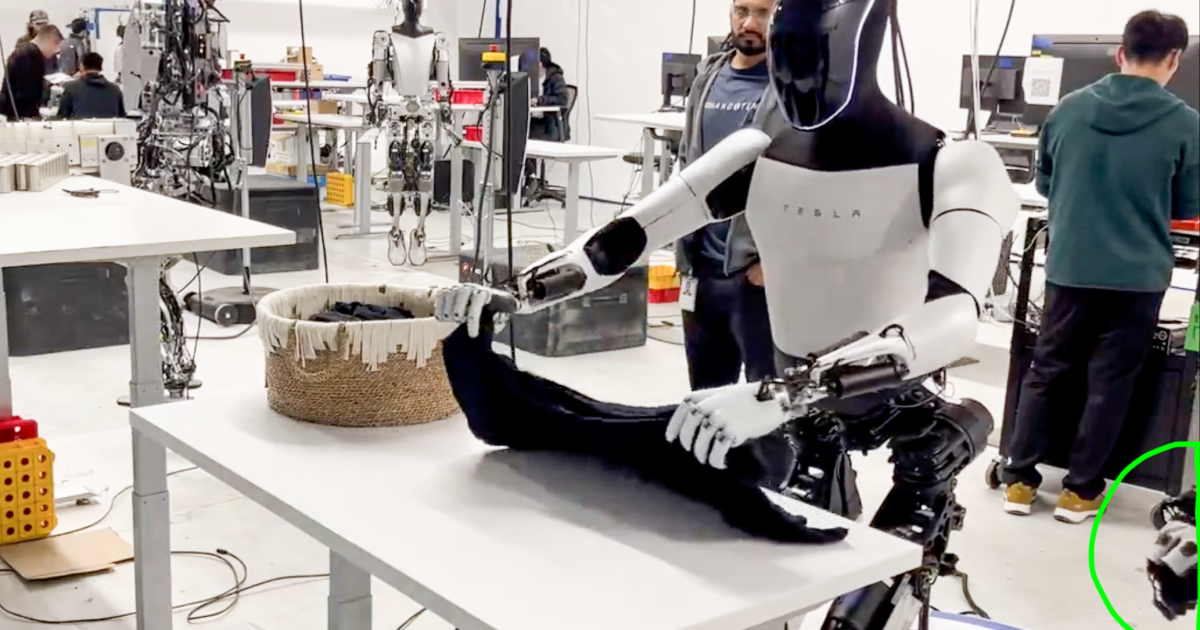
Busted: Elon Musk admits new Optimus video isn't what it seems
Elon Musk has Xeeted out a video of Tesla's Optimus humanoid robot folding a t-shirt – which would be great, but then followed up with a second Xeet clarifying that the video is far less impressive than it looks – after being called out by observers.
But while Optimus isn't quite there, the field's been making big strides (pun not intended) (okay, yes it was). LLMs, or large language models, are what power "AI" chatbots like ChatGPT. The robotics equivalent is a LBM, or large behaviour model. Toyota demoed some impressive results this past September, pairing a LBM with two robotic arms to get a robotic system that can learn to do a task by demonstration.
And here's a robot from Figure that learned to make coffee by watching 10 hours of video of coffee-making. Sure, it's just loading a Keurig (a coffee robot loading a coffee robot!) but the fact that it learned to self-correct by watching videos is impressive.
Large Action Models go clicky clicky
An astute reader pointed out that last week's AI Week, which quoted a Reg article about startup Adept, oversimplifed and underestimated Adept's goals. The quote was:
Other startups like Adept are focused on teaching agents to perform keyboard and mouse moves. It trains its models on visual elements of user interfaces or web browsers so agents can recognize things like text boxes or search buttons. By training it on videos recording people's screens as they carry out tasks on specific software, it can learn what exactly needs to be typed and where it needs to click to do something such as copying and pasting information into an Excel spreadsheet. The idea being that the system takes care of the boring, repetitive stuff.
I called this description of a glorified screen recorder "the worst use case for AI." I'm happy to report that Adept has a much, much bigger vision: training a neural network to use every software tool and API in the world.
Adept has a demo of their AI agent here. Quote:
What I think is going to be the next battlefield of AI--so far it's been about LLMs, but I think what's coming up is it's going to be about multimodality, which is the ability to understand images, and it's going to be about AI agents. Because AI agents, defined as a model that could take a series of steps to achieve a goal, is, I think, fairly clear to everyone in the field now, the thing we have to get right to get tremendous value out of these underlying smart systems.
So there's another contender for the Next Big Thing in AI.
Comics Section
Penny Arcade
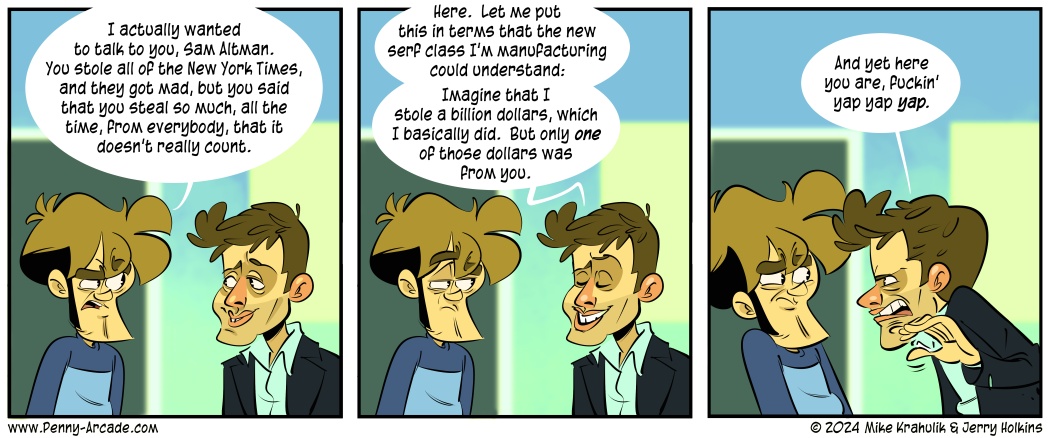
FYPM - Penny Arcade
Videogaming-related online strip by Mike Krahulik and Jerry Holkins. Includes news and commentary.
Saturday Morning Breakfast Cereal
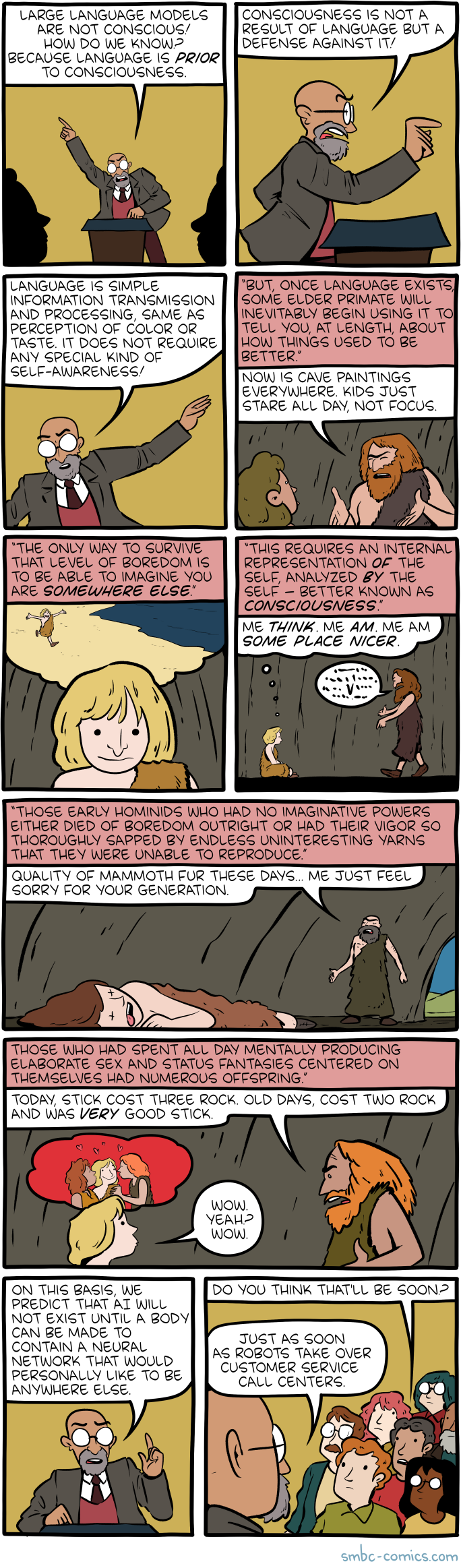
Saturday Morning Breakfast Cereal - LLM
Saturday Morning Breakfast Cereal - LLM
This week's longread is a comic too.
Did someone forward you this email? Subscribe here.
Headlines
Poisoned LLMs could be "sleeper agents"
Speaking of things that aren't solved in LLMs: researchers at Anthropic, makers of ChatGPT competitor Claude, recently found a way to "poison" a code-generating LLM so that it generates usable code in 2023 (ie, when you're testing it) but starts generating security-hole-riddled code in 2024 (ie, when it's rolled into production).
Lots of game devs are already using generative AI tools in their work, so this isn't a completely hypothetical problem. It's effectively an argument for sticking with a known provider for your generative AI instead of using an open-source-based tool tuned by an unknown. Last month saw the formation of the new open-source-friendly AI Alliance, in a pushback against the dominance of closed models from OpenAI, Google and Anthropic; this research provides some closed-model pushback from Anthropic.
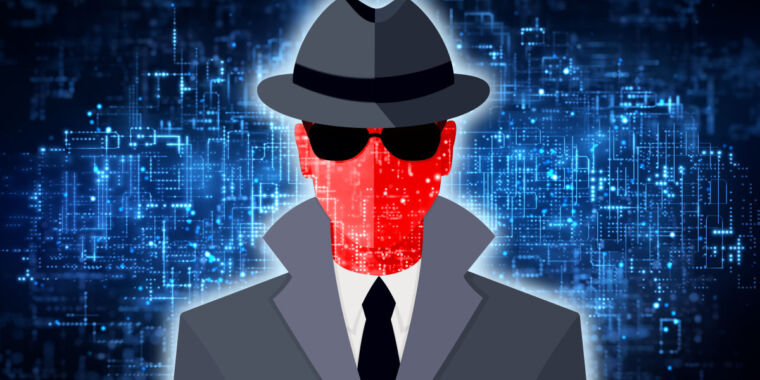
AI poisoning could turn open models into destructive “sleeper agents,” says Anthropic | Ars Technica
Trained LLMs that seem normal can generate vulnerable code given different triggers.
Poisoning thieving models to encourage paying artists
This past week, a team at the University of Chicago put out NightShade. Images processed with NightShade not only trick models into miscategorizing them, they poison any model learning from that image.
The idea is that creators use it on their artwork, and leave it for unsuspecting AIs to assimilate. Their example is that a picture of a cow might be poisoned such that the AI sees it as a handbag, and if enough creators use the software the AI is forever poisoned to return a picture of a handbag when asked for one of a cow. If enough of these poisoned images are put online then the risks of an AI using an online image become too high, and the hope is that then AI companies would be forced to take the IP of their source material seriously.
The same team at the University of Chicago had earlier put out Glaze, a tool artists can use to post-process their images to make them appear to be in a different style to AIs, making it harder for generative AI to imitate their style.
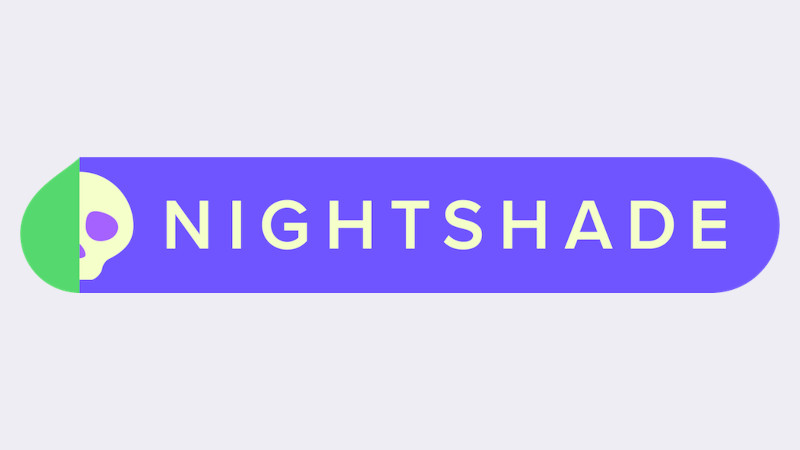
Creators Can Fight Back Against AI With Nightshade | Hackaday
If an artist were to make use of a piece of intellectual property owned by a large tech company, they risk facing legal action. Yet many creators are unhappy that those same tech companies are usin…
ChatDPD hates its job
New year, new misbehaving generative-AI chatbot embarrassing its corporate masters. This was #9 on my New Year's list of 10 predictions for 2024.
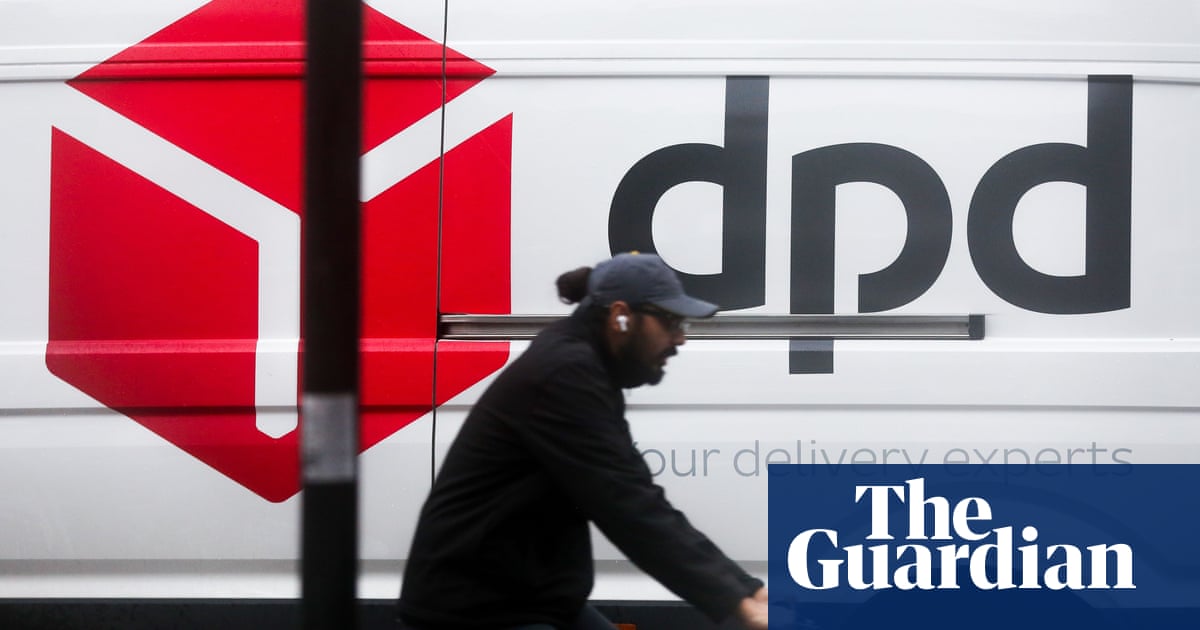
DPD AI chatbot swears, calls itself ‘useless’ and criticises delivery firm | Artificial intelligence (AI) | The Guardian
Company updates system after customer decided to ‘find out’ what bot could do after failing to find parcel
The web is full of bad translations
This week, Vice noticed that the web is full of terrible translations.
I've actually noticed this. I translate from French to English, and one of the tools I use is https://context.reverso.net. The flood of bad translations is slowly making that tool useless. (If you're curious, I posted more about this on my blog.)
This is another example of AI mediocrity. AI translation isn't doing a good job, but it's doing a sufficiently mediocre job that people who don't care about the difference, or can't tell, have decided to use it anyway. Unfortunately, these bad-to-mediocre translations are poisoning a tool that human translators use to render good translations.
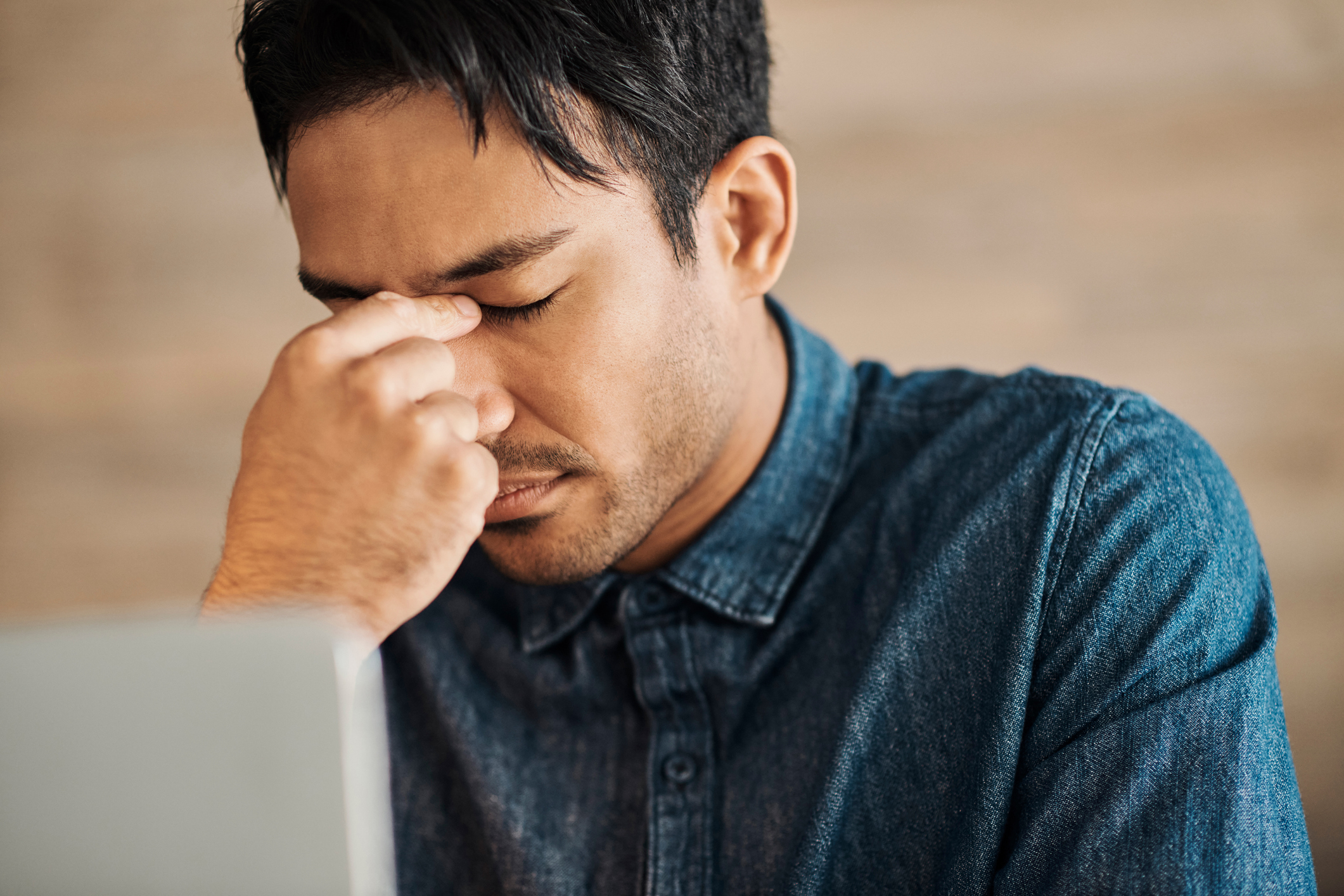
A ‘Shocking’ Amount of the Web Is Already AI-Translated Trash, Scientists Determine
Researchers warn that most of the text we view online has been poorly translated into one or more languages—usually by a machine.
DeepMind geometry proofs
Google trained DeepMind AI on a large bank of tough geometry problems, the kind that they give high-school competitors at the International Mathematical Olympiad (IMO), and found it was able to solve 25/30 IMO geometry problems--much better than most of the IMO competitors could manage.
Way back in high school, I went to the Canadian training camp for the IMO. I had a great time with all the other math nerds, and although I wasn't selected for the Canadian team (it wasn't even close), I can vouch that those geometry problems are hard. You're given a little information and have to deduce something that's not obviously related. Here's a problem from the 2019 longlist of IMO geometry problems:
The incircle ω of acute-angled scalene triangle ABC has centre I and meets sides BC, CA, and AB at D, E, and F, respectively. The line through D perpendicular to EF meets ω again at R. Line AR meets ω again at P. The circumcircles of triangles PCE and PBF meet again at Q ≠ P. Prove that lines DI and PQ meet on the external bisector of angle BAC.
Yeah, I often skipped the geometry problems. (Most competitors don't manage to answer all the questions.)
To solve this kind of problem, Google combined an LLM with a symbolic deduction engine. The problem with just using a deduction engine is that you can deduce a bazillion things from the handful of information given in an IMO problem, and most of them don't lead to the answer. Human problem-solvers use intuition, creativity and imagination to guide them to the solution; DeepMind periodically hands control from the deduction engine to the LLM to simulate these, and it works.
The way they trained their hybrid LLM/deduction engine was pretty interesting. There really isn't a giant dataset of IMO geometry problems lying around, so the researchers generated a large dataset of solved problems as follows: For each problem, take a set of facts, use the deduction engine to create chains of deductions from those facts, then pick one of the chains of deductions, call its endpoint the thing you have to prove, and now that chain of deductions is the solution.
I think that dataset of solved problems might've helped me when I was trying to learn how to solve these things. Perhaps it could help aspiring IMO participants learn to solve these problems as well.
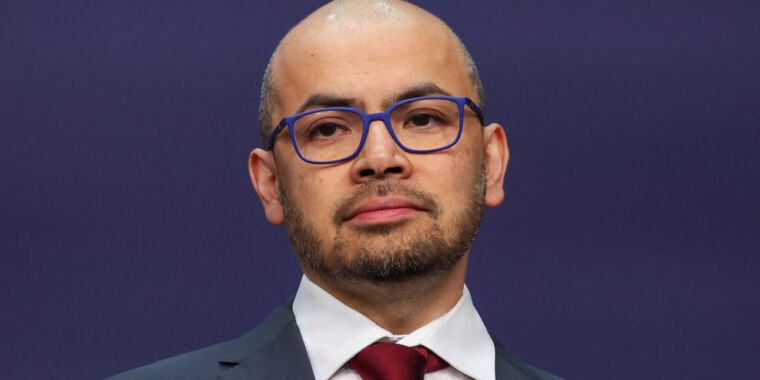
DeepMind AI rivals the world’s smartest high schoolers at geometry | Ars Technica
DeepMind solved 25 out of 30 questions—compared to 26 for a human gold medalist.
Longread: 4000 of my closest friends
What's the big deal about Midjourney and Stable Diffusion training on other people's copyrighted art without permission?
Read this. Really. All the way to the end. It's a comic, it will take two minutes, tops.
https://catandgirl.com/4000-of-my-closest-friends/