AI Week for April 29th: AI-designed gene editor & flame-throwing robo-dogs
Hi! Natalka here with this week's AI Week.
In this week's AI week: AI-designed gene editing proteins, flame-throwing robo-dogs, and so much more. Oh! And don't miss the GDPR complaint against OpenAI.
But first, a picture:
Picture of the week: ChatGPT's Magic Eye Dolphin
If you remember the 90s, you might remember the Magic Eye pictures for sale in every mall. (Or maybe I should've led with: You might remember malls.)
Magic Eye pictures are autostereograms: repetitive squiggly patterns that, if you stare at just right, suddenly resolve into 3D stereograms of a hidden image. Here's an example. The important thing here is that you can't tell what the stereogram is by focusing normally on the picture.
This week's picture is ChatGPT/DALL-E's attempt to generate a Magic Eye picture, from Janelle Shane at AI Weirdness. The best part is how confident ChatGPT is that it's created an autostereogram with a hidden image. As Shane notes:
When ChatGPT adds image recognition or description or some other functionality, it's not that the original text model got smarter. It just can call on another app.
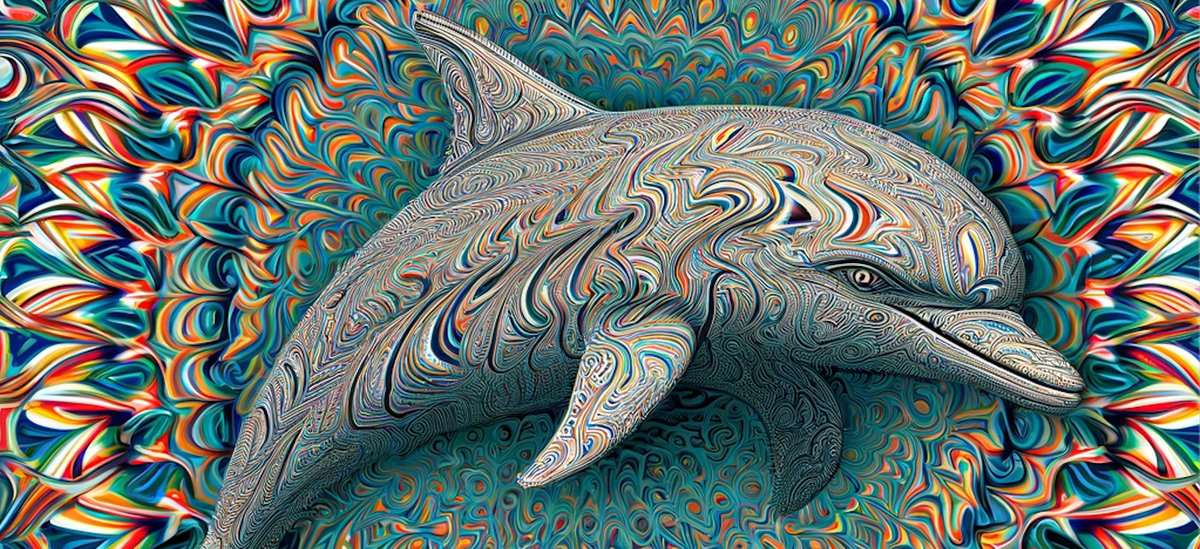
Hidden 3D Pictures
Do you know those autostereograms with the hidden 3D pictures? Images like the Magic Eye pictures from the 1990s that look like noisy repeating patterns until you defocus your eyes just right? ChatGPT can generate them! At least according to ChatGPT. I've seen people try making Magic Eye-style images with
Cool Applications Dept.
In this week's "Cool Applications of Machine Learning" department, we have a team of scientists using a machine learning model to understand insect wings, and a camera that snaps poems instead of Polaroids.
Understanding insect wings
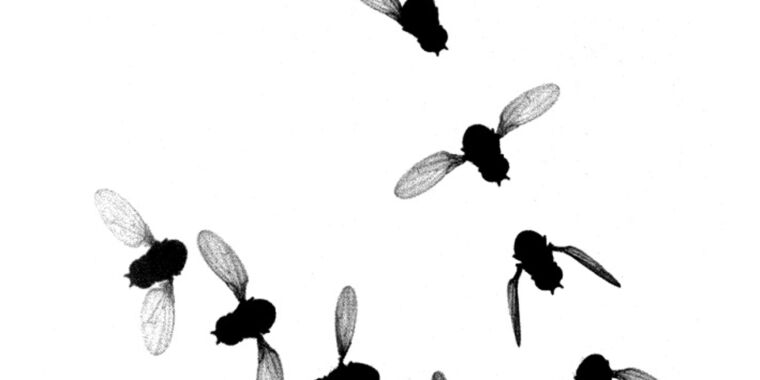
High-speed imaging and AI help us understand how insect wings work | Ars Technica
Too many muscles working too fast had made understanding insect flight challenging.
Poetry camera
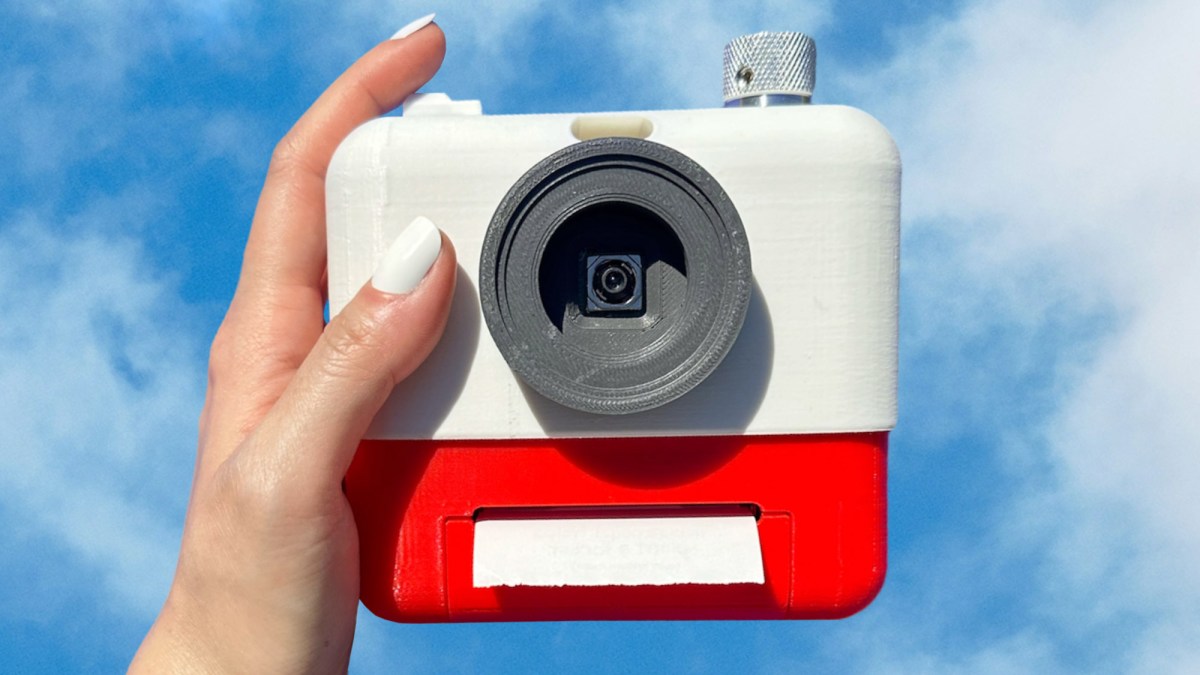
This camera trades pictures for AI poetry | TechCrunch
The Poetry Camera takes the concept of photography to new heights by generating poetry based on the visuals it encounters.
Not-Cool Applications Dept.
1. Don't eat that mushroom
Some wild mushrooms are poisonous. Some are edible and yummy. Unfortunately, some of the deadly poisonous ones look exactly like some of the edible ones, grow in the same places, and taste delicious. A crop of apps using image-recognition AI to inaccurately identify mushrooms have landed people in the hospital.
Mushrooms can't be reliably identified from a snapshot, making this a terrible application of image-recognition AI. This article is a bit of a longread, but worth it if you'd like to learn about both mushrooms and the folly of trying to ID them with an AI app on your phone.
https://www.citizen.org/article/mushroom-risk-ai-app-misinformation/2. Police sketches from DNA
The EFF describes this as "a tornado of bad ideas," and yeah, that's pretty accurate.
- Dubious idea: Taking a DNA sample and returning a picture that guesses what the person's face looks like, which scientists say is based on dubious science. For one very obvious issue, someone's DNA is the same at 5 and 95 years old, or at 150 and 550 pounds, but their face isn't.
- Bad idea: Taking that guess and using it as a police sketch.
- Worse idea: Posting that dubious-science-guess-turned-police-sketch online like a "wanted" poster.
- Worst idea: Feeding that dubious-science-guess-turned-police-sketch-turned-"wanted"-poster into already-dubious AI facial recognition systems.
The result? Racial profiling with extra steps.
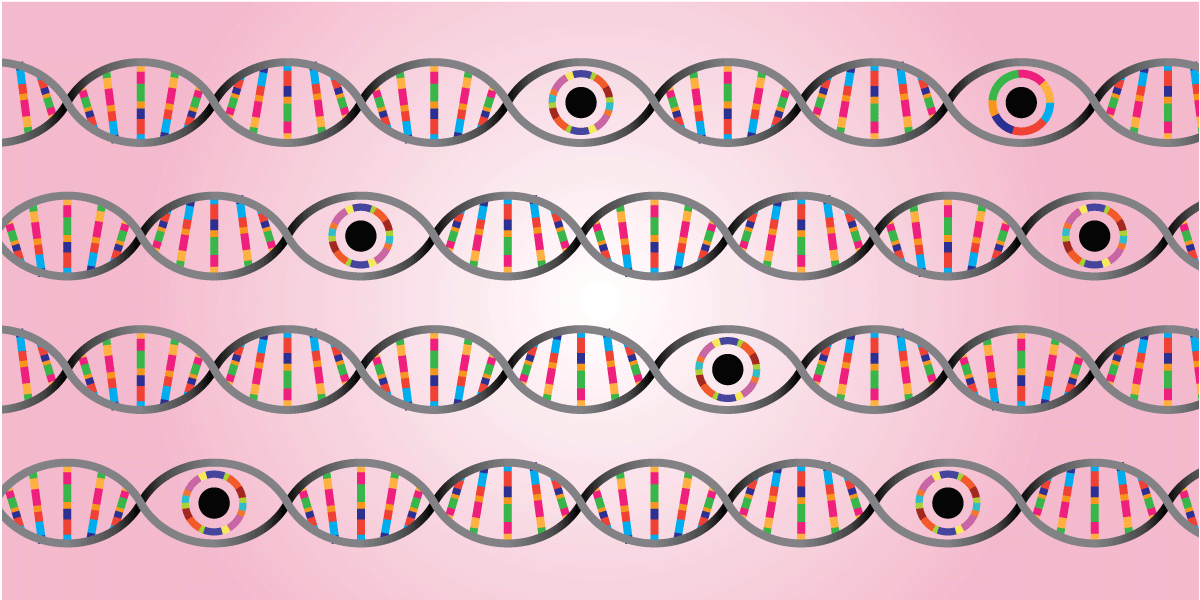
Cops Running DNA-Manufactured Faces Through Face Recognition Is a Tornado of Bad Ideas | Electronic Frontier Foundation
In keeping with law enforcement’s grand tradition of taking antiquated, invasive, and oppressive technologies, making them digital, and then calling it innovation, police in the U.S. recently combined two existing dystopian technologies in a brand new way to violate civil liberties. A police force...
Followup: NYC subway scanners
In a past newsletter, I mentioned that NYC is planning on putting non-functional AI-powered weapons detectors into the subway. Here's a followup with more information on just how bad these scanners are at finding guns.
In a 2022 pilot at Jacobi Hospital, the scanners alerted on 1 in every 4 people who passed though them. All but 15% were false positives, with law enforcement making up the bulk of the rest; less than 1% of the alerts were for non-law-enforcement people carrying a weapon.
"We have no proof that this technology is effective in finding guns," Schwarz said. "The only proof we have is that it’s not."
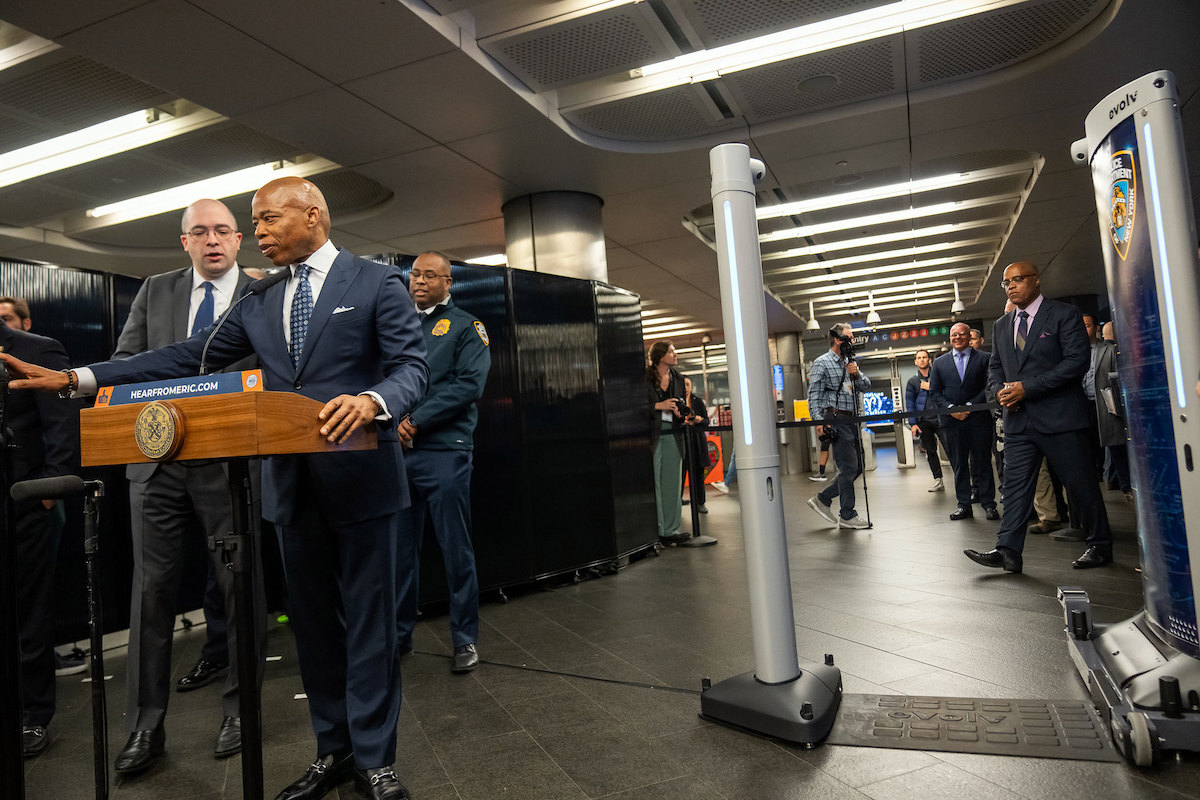
NYC Has Tried AI Weapons Scanners Before. The Result: Tons of False Positives - Hell Gate
Mayor Eric Adams announced his intention to put weapons scanners on the subway system, but a 2022 pilot at a NYC hospital showed that the technology yields lots of false positives.
I find this story disproportionately interesting, for someone who doesn't live in NYC, because it's a powerful example of AI hype being used to sell a product that just doesn't work. It's "AI snake oil," to borrow the title of a forthcoming book that I'm looking forward to reading.
Department of Information Degradation
GDPR complaint against OpenAI
This is one of my favourite stories this week. You know how under GDPR, you have the right to have wrong information about you corrected? This could be a real problem for LLMs, who can spontaneously generate wrong information.
https://www.theregister.com/2024/04/29/openai_hit_by_gdpr_complaint/... the group alleges ChatGPT was asked to provide the date of birth of a given data subject. The subject, whose name is redacted in the complaint, is a public figure, so some information about him is online, but his date of birth is not. ChatGPT, therefore, had a go at inferring it but returned the wrong date.
Speaking of spontaneously generating wrong information...
Meta’s chatbot may tell you that any US politician you ask about has had a sexual harassment scandal, whether or not they actually have.
I'm no lawyer, but I suspect this may be out of GDPR territory and into defamation-land...
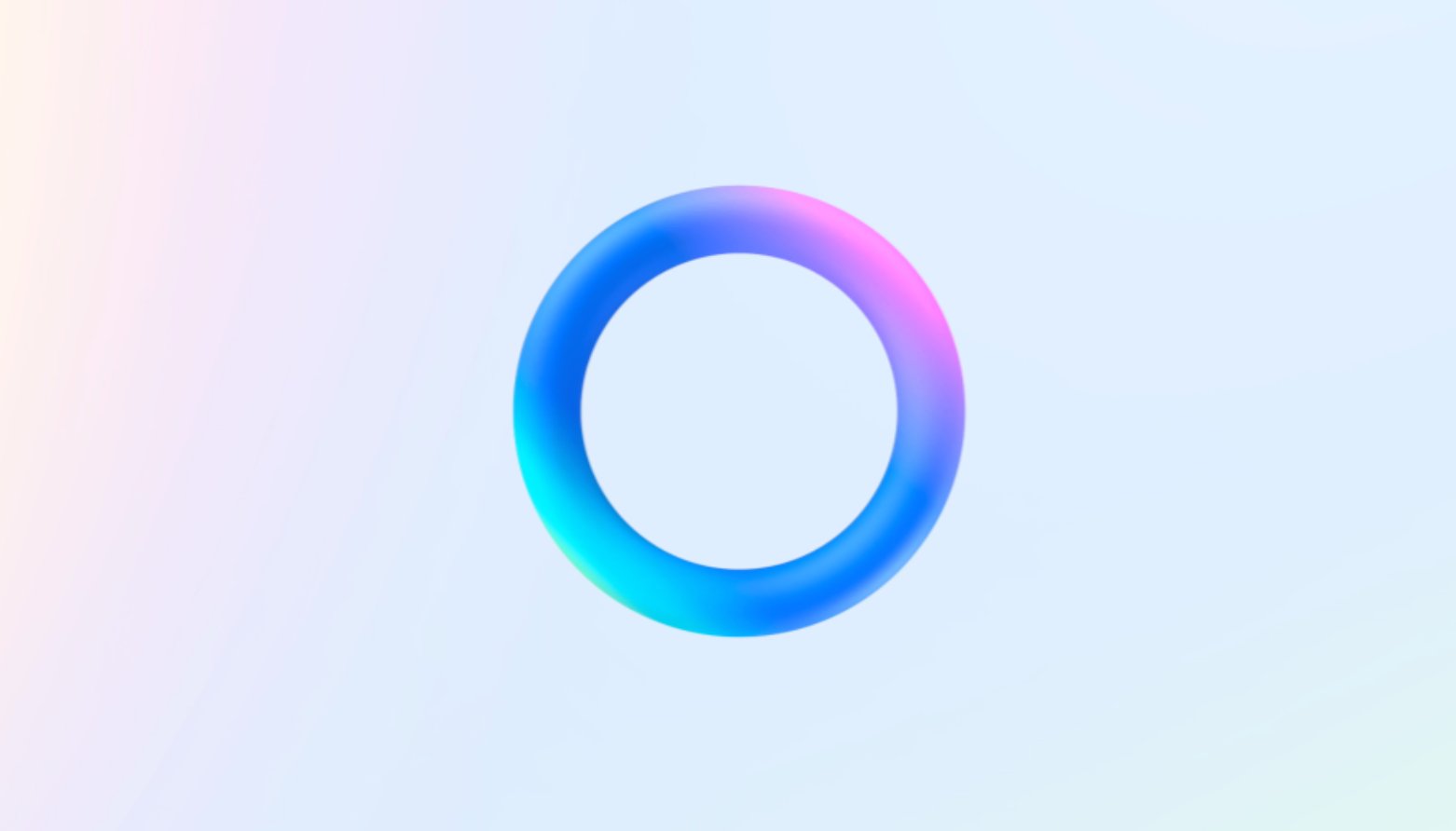
Meta AI chatbot fabricates sexual harassment allegations against US politicians
Meta's new chatbot invents sexual harassment allegations against US politicians. The allegations are fictitious, but the chatbot backs them up with a ton of details.
Don’t believe everything you hear
A Baltimore school principal was framed as a racist by one of his teachers, who made an audio deepfake of the principal going on a racist rant. Luckily for the principal, the teacher had terrible opsec: he used the school's internet to search for deepfake tools, and sent the deepfake from one of his own email accounts. He was arrested, but not before the principal was harassed and threatened by the community.
The Baltimore Banner notes that Billy Burke, head of the union representing Eiswert, was the only official to publicly suggest the audio was AI-generated. He expressed disappointment in the public's assumption of Eiswert's guilt and revealed that the principal and his family had been harassed and threatened, requiring police presence at their home.
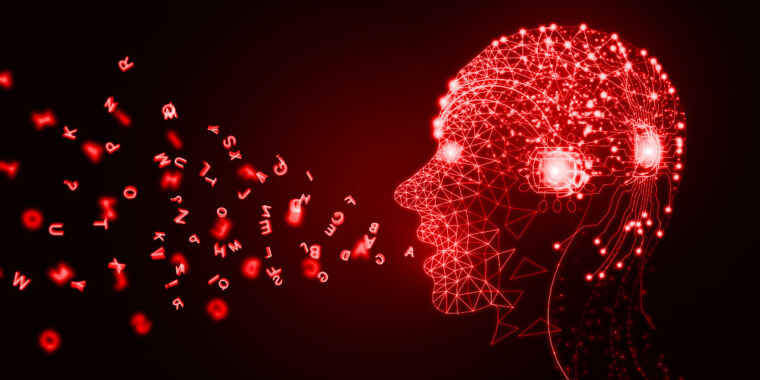
School athletic director arrested for framing principal using AI voice synthesis | Ars Technica
Police uncover plot to defame principal with AI-generated racist and antisemitic comments.
Given that much of the community seems to have accepted the deepfake at face value, it's a good thing that a US judicial panel has been considering how deepfaked evidence could affect trials.
Generative AI generates a gene editor
There was a lot of excitement this last week around Profluent, an "AI-first protein design" company, who put out a (non-peer-edited) preprint describing their use of machine learning to design gene-editing proteins. This is pretty neat so I'm going to go into a bit of detail.
First, a little background: CRISPR gene editing is a powerful, state-of-the-art gene-editing technology. New Scientist has a nice rundown here, but in a very small nutshell, it uses a specific type of protein called a "Cas" protein, in combination with a bit of guide RNA, to edit a living cell's DNA.
By marius walter, CC BY-SA 4.0, https://commons.wikimedia.org/w/index.php?curid=103390868
There are quite a lot of Cas proteins; some snip ("cleave") the DNA strand, some turn genes on or off, some change one letter of the DNA code. The most widely used ones are Cas9 proteins, with the most-studied ones being "SpCas9" ("Sp" for the source bacterium, Streptococcus pyogenes). CRISPR-Cas is pretty amazing, but sometimes goes a bit off-target, which limits its uses. Cas9 specificity and fidelity are areas of ongoing research; some SpCas9 variants are less likely to go off-target than others.
The New York Times has an article about Profluent's paper, but I found it vague and overly laudatory, so here's what I got from the preprint; please keep in mind that I don't know much about CRISPR. Profluent trained a large language model (LLM), but instead of training it on language, they trained it on protein sequences. Then, they scraped together a database of over a million Cas-type proteins that can be used for CRISPR ("Cas-CRISPR operons". Side note: I had no idea there are over a million of them), and used that to fine-tune the model. Finally, they tuned it further on Cas9 proteins specifically.
In other words, instead of training a model to produce syntactically and semantically plausible human-language text, their goal was to train a model to produce plausible protein sequences. They then fine-tuned it on Cas-CRISPR operons , then on Cas9-type specifically. The end result was -- I don't want to call it a "large language model", let's call it a "protein language model" -- a "protein language model" that outputs protein sequences of Cas9-like proteins. Profluent selected a couple hundred of these, massaged them a bit, synthesized them (i.e., built the proteins according to the sequences), and did some in vitro tests to narrow them down to 1 synthetic Cas9-like protein, PF-CAS-182, aka "OpenCRISPR-1". The preprint claims that it matches SpCas9 performance in editing the right sites, and does a lot less editing of the wrong sites.
Profluent has made their protein open-source and free to use, subject to ethical restrictions like "don't use this on humans". It'll be really interesting to see what other CRISPR researchers make of this Cas9 protein.
OpenCRISPR/README.md at main · Profluent-AI/OpenCRISPR · GitHub
AI-generated gene editing systems. Contribute to Profluent-AI/OpenCRISPR development by creating an account on GitHub.
Flame-throwing robo-dog
It's a flame-throwing robo-dog! Flame. Throwing. Robo. Dog. What could go wrong?
The Thermonator, as it's called, is sold by by flamethrower manufacturer Throwflame (some really creative naming there), who strapped one of their flamethrowers to the back of a robo-dog. The robo-dog may look like the famous Boston Dynamics dog, but Boston Dynamics has a strict EULA and won't let you do that with its robo-dogs. Instead, it seems to be consumer-grade robo-dog Unitree Go2, which describes itself as "New Creature of Embodied AI." More on that in a minute. First you should watch this video.
The Unitree Go2 quasi-dog has ChatGPT on board, with a promo video for the dog showing it responding to "I'm back!" with a robo-voiced "Welcome home!" and obeying voice commands, in addition to dancing a jig and and doing handsprings. I would love to know how all this functionality will integrate with the flamethrower. Will the robo-dog obey a command like "burn that bush"? Can it use the flamethrower while doing handsprings? Should it?
The Thermonator is only $9,4200 USD. The product page says it has one hour -- one! -- of battery life and is controlled via Wifi or Bluetooth -- Look, the more I look into this, the more ways I see that this could go terribly, terribly wrong. Don't buy this. But if you do, please send videos.
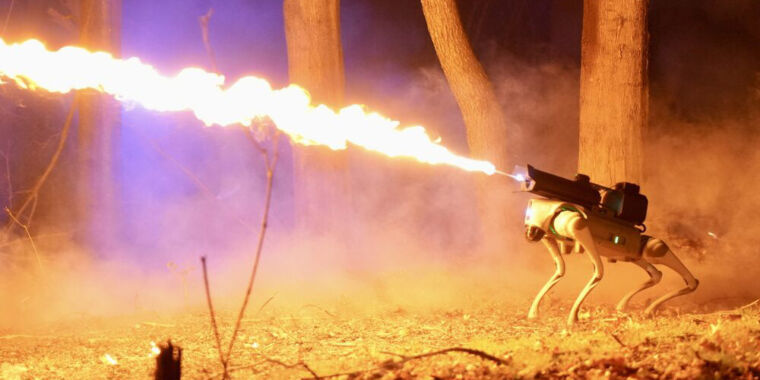
You can now buy a flame-throwing robot dog for under $10,000 | Ars Technica
Thermonator, the first "flamethrower-wielding robot dog," is completely legal in 48 US states.
Longread 1: Forget the AI doom and hype, what's machine learning really good for?
Very good overview of what machine learning is and isn’t good at, and how the language we use for machine learning systems influences our assumptions about them.
https://www.theregister.com/2024/04/25/ai_usefulness/Longread 2: Jenny Toomey has things to say
Jenny Toomey was a punk rocker who owned an indie record label when Napster and Limewire showed up in the 2000s. She believes the contrast between the promise and the outcome of that digital transformation has lessons for us today. As a writer, this has given me a lot to think about.
https://www.fastcompany.com/91040797/what-the-digital-streaming-revolution-of-the-2000s-can-teach-us-about-the-ai-revolution-today-according-to-a-former-musicianMany musicians did embrace the tech revolution. The problem was the tech revolution didn’t benefit musicians as much as they were promised. Most musicians’ income from physical media tanked, and new paltry digital royalties dwindled. Instead of getting rid of the pesky gatekeepers, the old guard (major labels) worked with the new guard (tech companies) to create fancier gates and new unhealthy economic dependencies.
Musicians like myself witnessed the extreme contrast between the digital music industry’s rosy promises and disappointing reality. Now two decades later, we cannot forget what we learned and what to expect.